Multi-data-center energy-saving routing method and system based on reinforcement learning
A multi-data center and reinforcement learning technology, applied in digital transmission systems, transmission systems, data exchange networks, etc.
- Summary
- Abstract
- Description
- Claims
- Application Information
AI Technical Summary
Problems solved by technology
Method used
Image
Examples
Embodiment 1
[0050] Such as figure 1 As shown, Embodiment 1 of the present disclosure provides a multi-data center energy-saving routing method based on reinforcement learning, including the following steps:
[0051] In the multi-data center network topology based on software-defined network architecture, for elastic transmission requirements, under the premise of ensuring load balancing, use reinforcement learning to find a topology subset that meets both low energy consumption and low bandwidth costs;
[0052]Using the nonlinear characteristics of the Chebyshev scalar function, optimize the optional action set in reinforcement learning, obtain the scalarized ε greedy strategy and replace the original action selection strategy, so as to solve the weight of energy consumption and bandwidth cost problem, obtain a high-quality solution set;
[0053] After the topology subset is finally selected, unselected link devices can be turned off, so as to meet transmission requirements at low cost a...
Embodiment 2
[0090] Embodiment 2 of the present disclosure provides a multi-data center energy-saving routing system based on reinforcement learning, including:
[0091] The node set acquisition module is configured to: for any transmission requirement, initialize the current transmission location as the starting point of the route, and obtain an optional node set for the next transmission;
[0092] The node selection module is configured to: select the next node from the set of optional nodes using a scalarized ε greedy strategy;
[0093] The node confirmation module is configured to: obtain the transition probability from the current node to the next node according to the link congestion factor, judge whether to select the node as the next node according to the comparison between the transition probability and the preset threshold, and if not, continue the node selection, If so, the selected node is used as the new routing starting point to continue routing node selection until the trans...
Embodiment 3
[0096] Embodiment 3 of the present disclosure provides a medium on which a program is stored, and when the program is executed by a processor, the steps in the reinforcement learning-based multi-data center energy-saving routing method described in Embodiment 1 of the present disclosure are implemented.
[0097] The detailed steps are the same as the reinforcement learning-based multi-data center energy-saving routing method provided in Embodiment 1, and will not be repeated here.
PUM
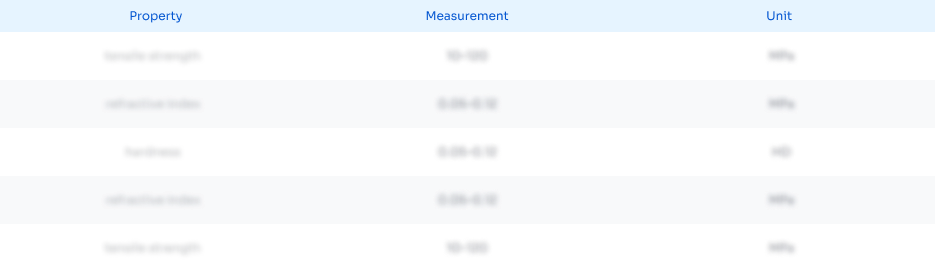
Abstract
Description
Claims
Application Information
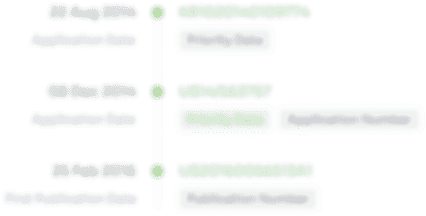
- R&D Engineer
- R&D Manager
- IP Professional
- Industry Leading Data Capabilities
- Powerful AI technology
- Patent DNA Extraction
Browse by: Latest US Patents, China's latest patents, Technical Efficacy Thesaurus, Application Domain, Technology Topic, Popular Technical Reports.
© 2024 PatSnap. All rights reserved.Legal|Privacy policy|Modern Slavery Act Transparency Statement|Sitemap|About US| Contact US: help@patsnap.com