Multi-modal machine translation method based on variational reasoning and multi-task learning
A multi-task learning and machine translation technology, applied in multi-modal machine translation based on variational reasoning and multi-task learning, in the field of machine translation, can solve problems such as not being effective, improve machine translation and reduce computational complexity Effect
- Summary
- Abstract
- Description
- Claims
- Application Information
AI Technical Summary
Problems solved by technology
Method used
Image
Examples
Embodiment
[0121] The following is the implementation process of this embodiment:
[0122] 1, such as figure 1 As shown, first, the original data such as images and texts are preprocessed accordingly. Image preprocessing includes denoising, normalization, etc., and text preprocessing includes word piece segmentation, word embedding, etc., which are input to the RNN text as training data Feature Encoder and VGG-16 Image Feature Encoder.
[0123] 2. After obtaining the data, first specify the hyperparameters such as the learning rate, then initialize the parameters of the variational multimodal machine translation model, and finally learn these parameters.
[0124] 3. Get a new image or text data, and do corresponding preprocessing as the training data, as the test data.
[0125] 4. Use a zero-matrix mask for other modalities, input the mask and the test data extracted above into the model, and use the previously learned variational multimodal machine translation model to obta...
PUM
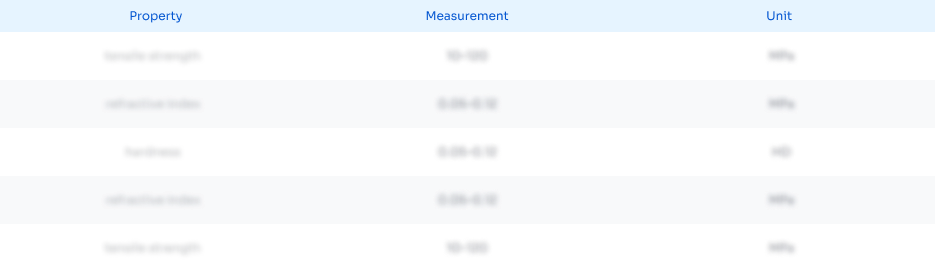
Abstract
Description
Claims
Application Information
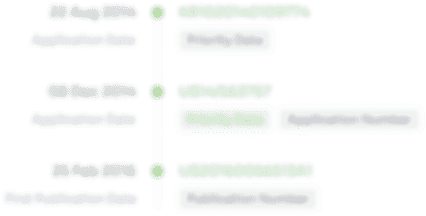
- R&D Engineer
- R&D Manager
- IP Professional
- Industry Leading Data Capabilities
- Powerful AI technology
- Patent DNA Extraction
Browse by: Latest US Patents, China's latest patents, Technical Efficacy Thesaurus, Application Domain, Technology Topic, Popular Technical Reports.
© 2024 PatSnap. All rights reserved.Legal|Privacy policy|Modern Slavery Act Transparency Statement|Sitemap|About US| Contact US: help@patsnap.com