Machine learning method based on difficult sample learning and neural network fusion
A neural network and sample learning technology, applied in neural learning methods, biological neural network models, integrated learning, etc., can solve problems such as difficult learning accuracy and difficult sample learning, and achieve accurate fusion results
- Summary
- Abstract
- Description
- Claims
- Application Information
AI Technical Summary
Problems solved by technology
Method used
Image
Examples
Embodiment 1
[0036] When the method of the present invention is specifically implemented, the neural network fusion is not limited to that described in step 1 and step 2, that is, neural network B 1 Not necessarily based on hard sample training; neural network A 1 with neural network B 1 Can be two separate networks obtained by other means;
[0037] Further, during specific implementation, the number of networks can be increased to more than two, and the network structures participating in the fusion can also be different, for example: "In the incremental learning, the network learned on the newly added training samples is fused with the original network, Or the situation of merging the networks obtained by two different learning methods is also a specific implementation derived from the method of the present invention.
[0038] This embodiment describes the specific implementation of learning the twin U-shaped neural network (hereinafter referred to as the collaborative segmentation net...
Embodiment 2
[0051] It should be noted that image 3 It is only a kind of neural network combination method, and other methods of combining the output results of two neurons into one are also applicable to the method of the present invention, including the following sub-steps:
[0052] Step 4): On all original samples, only for the co-segmentation network Learn the weights of all new neurons in the network to form a trained collaborative segmentation network
[0053] Step 5): Obtain the co-segmentation network Its structure and co-segmentation network and co-segmentation network have the same structure, and its parameters are the collaborative segmentation network learned in step 4 The weight of the newly added neuron in will be connected by the newly added neuron in the collaborative segmentation network co-segmentation network The result of the weighted combination of the parameters of the corresponding neurons.
[0054] So far, from step 1) to step 5), the first difficul...
Embodiment 3
[0069] The method can also be applied to solve the incremental learning problem of the neural network. After the neural network is learned on the existing training samples, when the training samples increase, the new neural network can only be learned on the newly added samples, and then the new neural network can be obtained by using the The above neural network fusion method fuses the new neural network with the existing neural network to obtain a refined neural network, which can not only adapt to the new data, but also maintain the calculation accuracy of the original data.
PUM
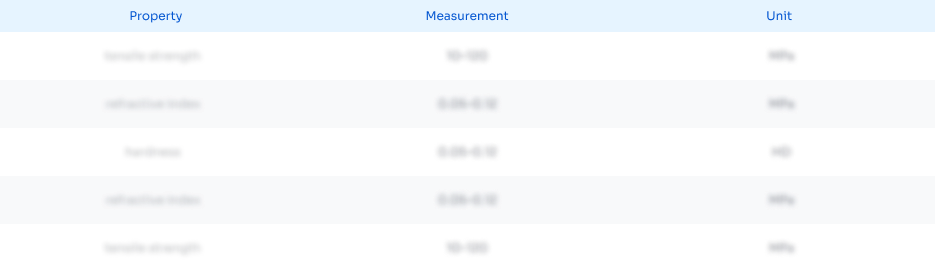
Abstract
Description
Claims
Application Information
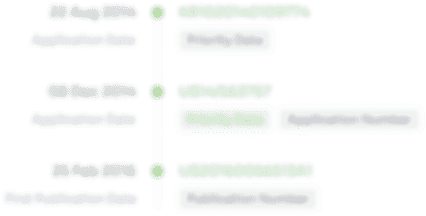
- R&D Engineer
- R&D Manager
- IP Professional
- Industry Leading Data Capabilities
- Powerful AI technology
- Patent DNA Extraction
Browse by: Latest US Patents, China's latest patents, Technical Efficacy Thesaurus, Application Domain, Technology Topic, Popular Technical Reports.
© 2024 PatSnap. All rights reserved.Legal|Privacy policy|Modern Slavery Act Transparency Statement|Sitemap|About US| Contact US: help@patsnap.com