Fixed-wing unmanned aerial vehicle cluster control collision avoidance method and device based on deep reinforcement learning
A reinforcement learning and unmanned aerial vehicle technology, applied in vehicle position/route/height control, control/adjustment system, non-electric variable control, etc., can solve the problem of unmanned aerial vehicle collision, and the rotorcraft control strategy cannot be directly applied Fixed-wing UAV swarm control, UAV large collision risk and other issues
- Summary
- Abstract
- Description
- Claims
- Application Information
AI Technical Summary
Problems solved by technology
Method used
Image
Examples
Embodiment Construction
[0058] The present invention will be further described below in conjunction with the accompanying drawings and specific preferred embodiments, but the protection scope of the present invention is not limited thereby.
[0059] Such as figure 1 , 2 As shown, the steps of the fixed-wing UAV cluster control collision avoidance method based on deep reinforcement learning in this embodiment include:
[0060] S1. Model training: establish a UAV kinematics model for generating UAV state data and D3QN (Dueling Double Deep Q-Network, competing dual Q-Network) for outputting UAV control instructions, and use various The historical interaction data during the interaction process between the wingman and the environment updates the network parameters of D3QN, and trains to form a D3QN model. -Leader aircraft joint state), according to the acquired state information (environmental state) of the wingman himself and the adjacent wingman, conduct situation assessment to assess the risk of col...
PUM
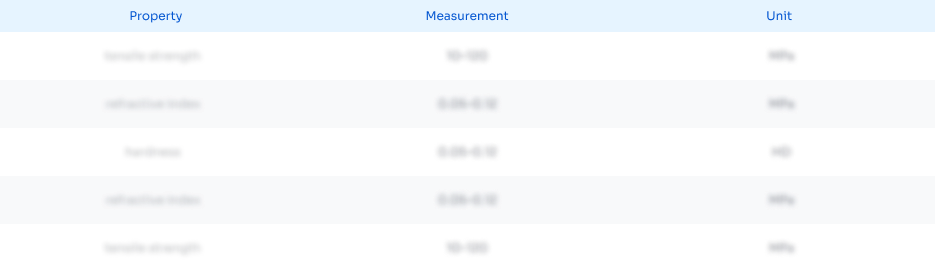
Abstract
Description
Claims
Application Information
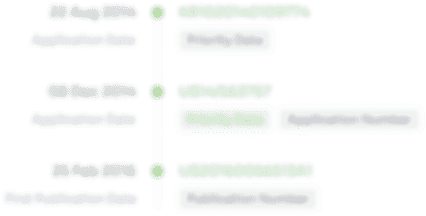
- Generate Ideas
- Intellectual Property
- Life Sciences
- Materials
- Tech Scout
- Unparalleled Data Quality
- Higher Quality Content
- 60% Fewer Hallucinations
Browse by: Latest US Patents, China's latest patents, Technical Efficacy Thesaurus, Application Domain, Technology Topic, Popular Technical Reports.
© 2025 PatSnap. All rights reserved.Legal|Privacy policy|Modern Slavery Act Transparency Statement|Sitemap|About US| Contact US: help@patsnap.com