Network abnormal traffic automatic detection method based on time sequence mining
A network anomaly and time series technology, applied in transmission systems, electrical components, etc., can solve problems such as cumbersome data processing modes, complex data collection methods, and complex network architectures
- Summary
- Abstract
- Description
- Claims
- Application Information
AI Technical Summary
Problems solved by technology
Method used
Image
Examples
Embodiment 1
[0094] Embodiment 1: Abnormal network traffic detection process
[0095] An automatic detection method for abnormal network traffic based on time series mining, such as figure 2 shown, including:
[0096] Step 1: Use the data import module to read the data value of the standard network traffic data. The standard network traffic data includes the data import template and the network traffic data exported by the third-party system, and then extract the core fields to form the initial time series model;
[0097] In step 1, the data import module can be developed in Java language. The data import template includes excel template, csv template, etc., and the template fields include: time stamp, total flow, inflow flow, outflow flow, and remarks. The data value is read, the core field is the time stamp t and the flow value v (including total flow, inflow flow, and outflow flow), and the read data is the network flow data value with time stamp, which is consistent with the time ser...
Embodiment 2
[0116] Example 2: Drill-down detection of abnormal network traffic
[0117] Step i: use the monthly abnormal data sequence detected by the automatic detection system for network abnormal traffic based on time series mining involved in the present invention as the input data to be detected, enter the data buffer space, and use the sliding window to divide the monthly data sequence into each day data subsequence;
[0118] Step ii: Use the fast learning method to learn the optimal front and back slack spaces, and obtain the optimal front and back slack spaces OPSRS for the abnormal subsequence of daily data. The operation process is consistent with the above step 4.
[0119] Step iii: Use the dynamic time warping method of the front and rear slack spaces to calculate the distance between the abnormal subsequences of the daily data, obtain the similarity matrix of the data subsequences, and then calculate the abnormality score of the data subsequences in the current data buffer sp...
PUM
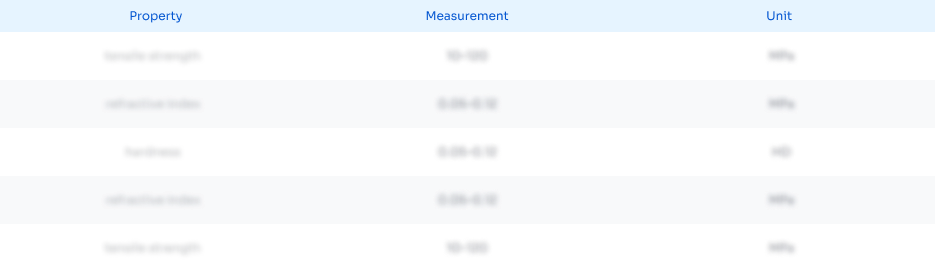
Abstract
Description
Claims
Application Information
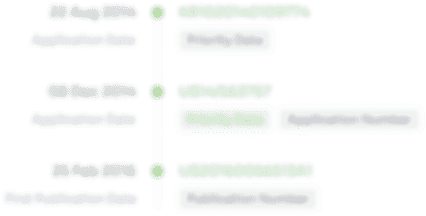
- R&D Engineer
- R&D Manager
- IP Professional
- Industry Leading Data Capabilities
- Powerful AI technology
- Patent DNA Extraction
Browse by: Latest US Patents, China's latest patents, Technical Efficacy Thesaurus, Application Domain, Technology Topic, Popular Technical Reports.
© 2024 PatSnap. All rights reserved.Legal|Privacy policy|Modern Slavery Act Transparency Statement|Sitemap|About US| Contact US: help@patsnap.com