Image data multi-label classification method
A classification method and image data technology, applied in the field of machine learning, can solve problems such as classification performance degradation
- Summary
- Abstract
- Description
- Claims
- Application Information
AI Technical Summary
Problems solved by technology
Method used
Image
Examples
Embodiment Construction
[0057] The collection of pictures to be classified D={pic i |1≤i≤n}, where n is the total number of pictures in the picture set D. In the picture set D, there are q ordered labels to be marked on each picture, and a total of l pictures have been marked whether they have the q labels, and the marked picture is pic i , 1≤i≤l.
[0058] (1) Calculate the weight matrix W and construct the graph relationship between pictures. This step includes the following sub-steps:
[0059] (1.1) Use the public dataset ImageNet on the website http: / / www.image-net.org / to train according to the method provided in 3.1 of the paper VERY DEEP CONVOLUTIONAL NETWORKS FOR LARGE-SCALE IMAGE RECOGNITION jointly published by Karen Simonyan and Andrew Zisserman in 2015 Change the convolutional neural network VGG Net proposed in this paper. VGG Net was jointly developed by the Visual Geometry Group of Oxford University and researchers from Google DeepMind, which can extract the feature vectors of the pic...
PUM
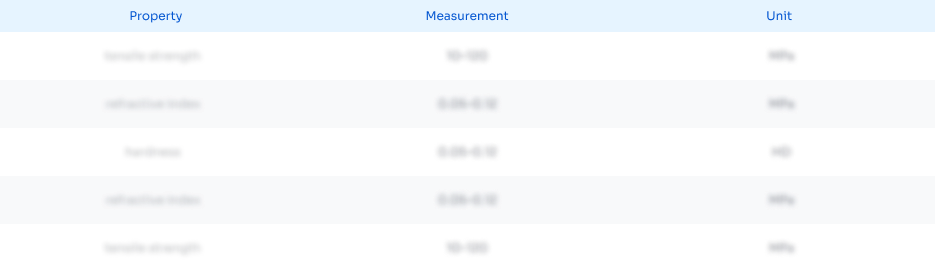
Abstract
Description
Claims
Application Information
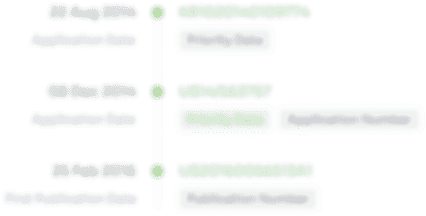
- R&D Engineer
- R&D Manager
- IP Professional
- Industry Leading Data Capabilities
- Powerful AI technology
- Patent DNA Extraction
Browse by: Latest US Patents, China's latest patents, Technical Efficacy Thesaurus, Application Domain, Technology Topic, Popular Technical Reports.
© 2024 PatSnap. All rights reserved.Legal|Privacy policy|Modern Slavery Act Transparency Statement|Sitemap|About US| Contact US: help@patsnap.com