Prediction model training method and device and electronic equipment
A prediction model and training method technology, applied in the field of machine learning, can solve the problems of high overhead cost, low prediction accuracy, low multi-value attribute support, etc., and achieve the effect of reducing overhead cost and simplifying deployment steps.
- Summary
- Abstract
- Description
- Claims
- Application Information
AI Technical Summary
Problems solved by technology
Method used
Image
Examples
Embodiment 1
[0068] The embodiment of the present application provides a training method of a prediction model, such as figure 1 As shown, the method includes: step S110 to step S140.
[0069] Step S110, acquiring sample data.
[0070] In this embodiment, the sample data may be obtained through custom editing, or collected from a terminal device. In practical applications, sample data is generally obtained from terminal devices. Specifically, the sample data may include user personal information corresponding to the terminal device, detailed information of applications installed on the terminal device, and the like. In actual application, the user's personal information may include age, gender, hobbies, etc.; the detailed information of the application program may represent the application program installed on the terminal device, such as QQ music, XX security guard, office, etc.
[0071] In practical applications, the terminal device may be electronic devices such as PCs, notebooks, mo...
Embodiment 2
[0114] The embodiment of the present application provides a training device for a predictive model, such as Figure 5 As shown, the device 50 may include: a data acquisition module 501, a vector conversion module 502, a model adjustment module 503 and a model training module 504, wherein,
[0115] A data acquisition module 501, configured to acquire sample data;
[0116] Vector conversion module 502, for utilizing the pre-built DeepFM model to convert sample data into corresponding vector data;
[0117] The model adjustment module 503 is used to obtain the position correspondence of any attribute feature in the vector data in the sample data, and store it in the DeepFM model to obtain the adjusted DeepFM model;
[0118] The model training module 504 is configured to use vector data to train the adjusted DeepFM model to obtain a DeepFM prediction model, so as to deploy the DeepFM prediction model.
[0119] In the embodiment of the present application, the sample data is obtai...
Embodiment 3
[0138] The embodiment of the present application provides an electronic device, such as Figure 6 as shown, Figure 6 The electronic device 600 shown includes: a processor 6001 and a memory 6003 . Wherein, the processor 6001 is connected to the memory 6003 , such as through a bus 6002 . Further, the electronic device 600 may further include a transceiver 6006 . It should be noted that in practical applications, the transceiver 6006 is not limited to one, and the structure of the electronic device 600 does not limit the embodiment of the present application.
[0139] The processor 6001 may be a CPU, a general processor, DSP, ASIC, FPGA or other programmable logic devices, transistor logic devices, hardware components or any combination thereof. It can implement or execute the various illustrative logical blocks, modules and circuits described in connection with the present disclosure. The processor 6001 may also be a combination that implements computing functions, for exam...
PUM
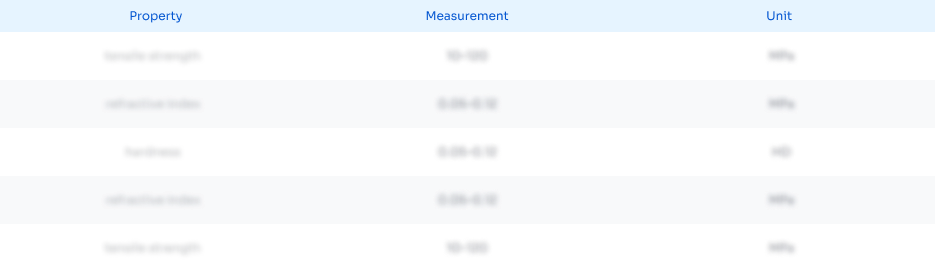
Abstract
Description
Claims
Application Information
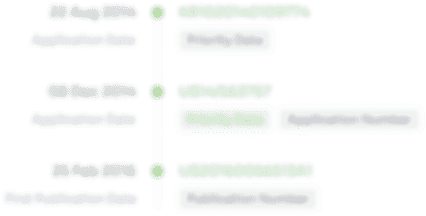
- R&D Engineer
- R&D Manager
- IP Professional
- Industry Leading Data Capabilities
- Powerful AI technology
- Patent DNA Extraction
Browse by: Latest US Patents, China's latest patents, Technical Efficacy Thesaurus, Application Domain, Technology Topic, Popular Technical Reports.
© 2024 PatSnap. All rights reserved.Legal|Privacy policy|Modern Slavery Act Transparency Statement|Sitemap|About US| Contact US: help@patsnap.com