An Intelligent Control Method of Adjacent State Bias for Jump System with Unknown Transition Probability
An intelligent control and transition system technology, applied in the field of engineering systems, can solve the problem that the transition probability information cannot be known in advance
- Summary
- Abstract
- Description
- Claims
- Application Information
AI Technical Summary
Problems solved by technology
Method used
Image
Examples
Embodiment 1
[0055] This embodiment provides an intelligent control method for adjacent-state deviation using an unknown transition probability jump system for the control problem of the DC motor jump circuit system in engineering technology. The method includes:
[0056] Step 1: Establish a discrete Markov jump system model of the DC motor jump circuit system. First, the motor dynamics of the DC jump circuit are described as:
[0057]
[0058]
[0059] Among them, r(t)=1, 2, 3 respectively represent the working states of the motor in three power modes: normal power, low power, and medium power. The system has different parameters in different working modes. t is the continuous time, u(t) is the total input voltage, i is the motor current, v(t) is the angular velocity of the motor; K m is the motor load constant, K e is the counter electromotive force constant, K d is the damping constant, R m is the total resistance of the circuit connection, L is the inductance; J is the mecha...
Embodiment 2
[0099] In this embodiment, aiming at the DC motor jump circuit, an intelligent control method for adjacent state deviation of an unknown transition probability jump system is provided for simulation experiment verification. In order to verify the effectiveness of the proposed method, the parameters of the discrete Markov jump system are described as follows :
[0100]
[0101] B 1 =B 2 =B 3 =(0 1) T ,
[0102]
[0103] D. 1 =D 2 =D 3 =(0 0 1) T , G 1 =G 2 =G 3 =(0 0.1) T .
[0104] Without loss of generality, the transition probability matrix is selected to obey the Gaussian random distribution, and the randomness is described by the following Gaussian probability density matrix:
[0105]
[0106] Among them, n(μ,σ) represents the Gaussian transition probability density function, μ is the mean, and σ is the variance.
[0107] Select the modal trajectory length K=20, the number of trajectories T=500, the weight rate λ=0.1, and the anti-interference pe...
PUM
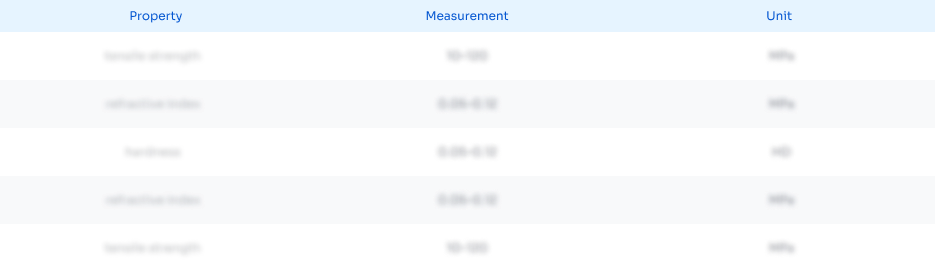
Abstract
Description
Claims
Application Information
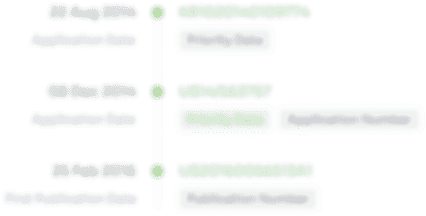
- R&D Engineer
- R&D Manager
- IP Professional
- Industry Leading Data Capabilities
- Powerful AI technology
- Patent DNA Extraction
Browse by: Latest US Patents, China's latest patents, Technical Efficacy Thesaurus, Application Domain, Technology Topic, Popular Technical Reports.
© 2024 PatSnap. All rights reserved.Legal|Privacy policy|Modern Slavery Act Transparency Statement|Sitemap|About US| Contact US: help@patsnap.com