Building operation energy consumption prediction method
A technology for operating energy consumption and forecasting methods, applied in forecasting, kernel methods, neural learning methods, etc., can solve problems such as complex factors, accumulation of combined model errors, and inability to meet optimal operations well, and achieve the effect of improving forecasting accuracy.
- Summary
- Abstract
- Description
- Claims
- Application Information
AI Technical Summary
Problems solved by technology
Method used
Image
Examples
Embodiment Construction
[0055] The invention provides a method for predicting energy consumption in building operation, which reconstructs the phase space of the time series of the research object, judges that it has chaotic characteristics, establishes a combination model of chaos theory and support vector regression for training, and uses Markov chain to eliminate the combination model Due to the cumulative error generated by the parameter transmission, the final prediction result is obtained to predict the energy consumption of the building.
[0056] see figure 1 , the present invention a kind of building operation energy consumption prediction method, comprises the following steps:
[0057] S1. Process the energy consumption data of office buildings;
[0058] The energy consumption of office buildings is processed and classified into water consumption, electricity consumption, gas consumption, central cooling / heating and other energy consumption.
[0059] S2. Carry out characteristic analysis o...
PUM
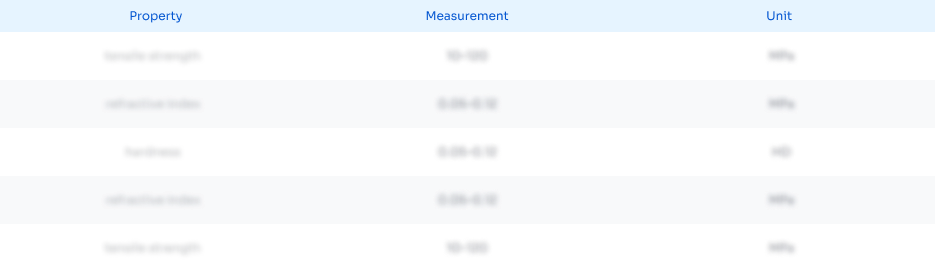
Abstract
Description
Claims
Application Information
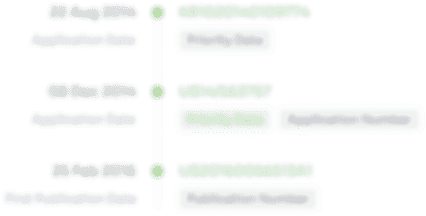
- R&D
- Intellectual Property
- Life Sciences
- Materials
- Tech Scout
- Unparalleled Data Quality
- Higher Quality Content
- 60% Fewer Hallucinations
Browse by: Latest US Patents, China's latest patents, Technical Efficacy Thesaurus, Application Domain, Technology Topic, Popular Technical Reports.
© 2025 PatSnap. All rights reserved.Legal|Privacy policy|Modern Slavery Act Transparency Statement|Sitemap|About US| Contact US: help@patsnap.com