Image reconstruction method based on iterative convolutional neural network
A convolutional neural network and image reconstruction technology, which is applied in the field of image reconstruction based on iterative convolutional neural network, can solve the problem that the image structure cannot be reconstructed, and achieve the effect of improving clarity, enriching image details, and clearing image edges
- Summary
- Abstract
- Description
- Claims
- Application Information
AI Technical Summary
Problems solved by technology
Method used
Image
Examples
Embodiment Construction
[0057] In order to enable those skilled in the art to better understand the technical solutions in this application, the following will clearly and completely describe the technical solutions in the embodiments of this application with reference to the drawings in the embodiments of this application. Obviously, the described The embodiments are only a part of the embodiments of the present application, not all the embodiments. Based on the embodiments in this application, all other embodiments obtained by those of ordinary skill in the art without creative work should fall within the protection scope of this application.
[0058] Combine figure 1 with image 3 As shown, the embodiment of this application discloses an image reconstruction method based on iterative convolutional neural network, including:
[0059] S101: Train a multi-scale progressive convolutional neural network to obtain a convolutional neural network model of angular image clusters.
[0060] Such as figure 2 with...
PUM
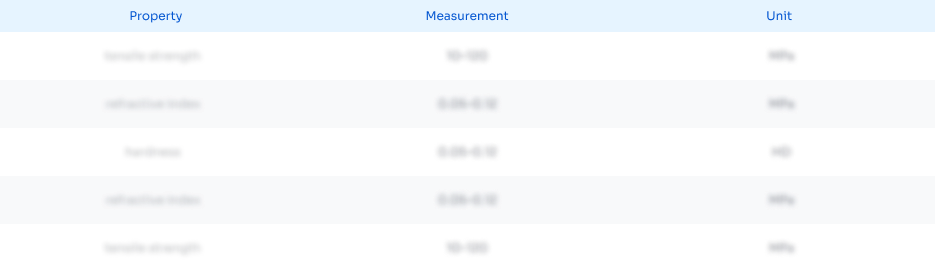
Abstract
Description
Claims
Application Information
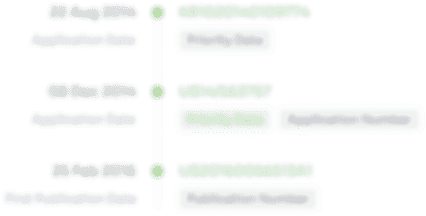
- R&D Engineer
- R&D Manager
- IP Professional
- Industry Leading Data Capabilities
- Powerful AI technology
- Patent DNA Extraction
Browse by: Latest US Patents, China's latest patents, Technical Efficacy Thesaurus, Application Domain, Technology Topic, Popular Technical Reports.
© 2024 PatSnap. All rights reserved.Legal|Privacy policy|Modern Slavery Act Transparency Statement|Sitemap|About US| Contact US: help@patsnap.com