Depth subspace clustering method and system for realizing effective feature extraction
A technology of feature extraction and clustering method, applied in the fields of instruments, character and pattern recognition, computer parts, etc., can solve the problems of inconsistent labels, no mutual promotion, unfavorable feature learning, etc., to avoid label misalignment and reduce noise data. The effect of improving the clustering effect
- Summary
- Abstract
- Description
- Claims
- Application Information
AI Technical Summary
Problems solved by technology
Method used
Image
Examples
Embodiment Construction
[0055]The following will clearly and completely describe the technical solutions in the embodiments of the present invention with reference to the accompanying drawings in the embodiments of the present invention. Obviously, the described embodiments are only some, not all, embodiments of the present invention. Based on the embodiments of the present invention, all other embodiments obtained by persons of ordinary skill in the art without creative efforts fall within the protection scope of the present invention.
[0056] The terms "comprising" and "comprising" indicate the presence of described features, integers, steps, operations, elements and / or components, but do not exclude the presence of one or more other features, integers, steps, operations, elements, components and / or The presence or addition of its collection.
[0057] The term "and / or" refers to any combination of one or more of the associated listed items and all possible combinations, and includes such combinati...
PUM
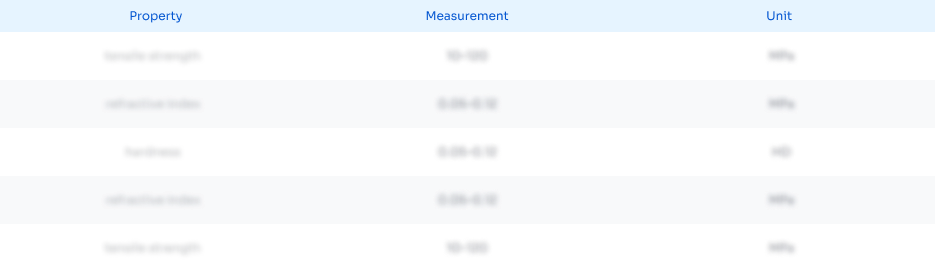
Abstract
Description
Claims
Application Information
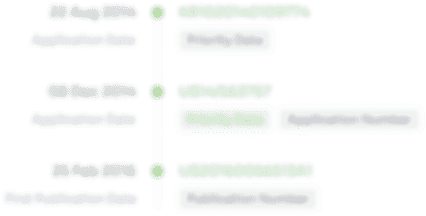
- R&D Engineer
- R&D Manager
- IP Professional
- Industry Leading Data Capabilities
- Powerful AI technology
- Patent DNA Extraction
Browse by: Latest US Patents, China's latest patents, Technical Efficacy Thesaurus, Application Domain, Technology Topic, Popular Technical Reports.
© 2024 PatSnap. All rights reserved.Legal|Privacy policy|Modern Slavery Act Transparency Statement|Sitemap|About US| Contact US: help@patsnap.com