Posterior probability algorithm based on weighted decision tree
A posterior probability and decision tree technology, applied in the direction of calculation, calculation model, special data processing application, etc., can solve problems such as inapplicability, increase reliability, reduce uneven distribution of data samples, and reduce overfitting. Effect
- Summary
- Abstract
- Description
- Claims
- Application Information
AI Technical Summary
Problems solved by technology
Method used
Image
Examples
Embodiment Construction
[0027] The present invention will be further explained below in conjunction with the accompanying drawings and specific embodiments.
[0028] like figure 1 As shown, a decision tree-based posterior probability algorithm, the method is based on the conventional decision tree algorithm, and when the decision tree is split, the weight factor is added to improve the influence of the confidence classification, thus strengthening the high confidence The final information entropy of the node, the changed information entropy will effectively change the overall probability distribution relationship, and untrustworthy classification will reduce its own influence;
[0029] The specific steps of the decision tree-based posterior probability algorithm are as follows:
[0030] The classification of data is converted into numerical value, and the processed data is used as the data source, and each classification situation is iteratively iterated, and the best information gain is calculated ...
PUM
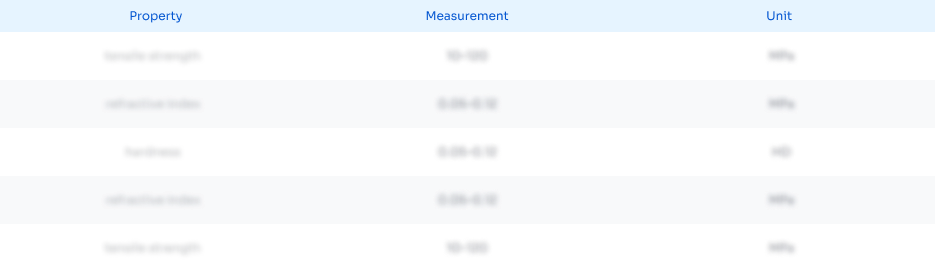
Abstract
Description
Claims
Application Information
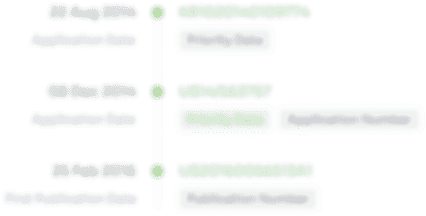
- R&D
- Intellectual Property
- Life Sciences
- Materials
- Tech Scout
- Unparalleled Data Quality
- Higher Quality Content
- 60% Fewer Hallucinations
Browse by: Latest US Patents, China's latest patents, Technical Efficacy Thesaurus, Application Domain, Technology Topic, Popular Technical Reports.
© 2025 PatSnap. All rights reserved.Legal|Privacy policy|Modern Slavery Act Transparency Statement|Sitemap|About US| Contact US: help@patsnap.com