Hyperspectral image denoising method based on multi-target low-rank sparsity and space spectrum total variation
A hyperspectral, total variational technology, applied in the field of remote sensing image processing, can solve the problems of high cost, affect the denoising accuracy of hyperspectral images, inaccurate sparse noise modeling, etc., and achieve high-precision hyperspectral image denoising. results, improve computational efficiency, and avoid the effect of modeling errors
- Summary
- Abstract
- Description
- Claims
- Application Information
AI Technical Summary
Problems solved by technology
Method used
Image
Examples
Embodiment Construction
[0049] The technical solutions of the present invention will be further specifically described below through the embodiments and in conjunction with the accompanying drawings.
[0050] A space-spectrum clustering method for remote sensing images based on a multi-objective sine-cosine algorithm, comprising the following steps:
[0051] Step 1, input a noisy analog image Washington DC Mall to be denoised, such as figure 1 As shown, the size of the image is 200×200×191, and it is pre-processed in blocks. Here, the size of each image block is 50×50×191. The following steps process each image block separately;
[0052] Step 2, modeling multiple objective functions for hyperspectral image denoising, constructing sparse noise items, low-rank image items, data fidelity joint space-spectrum full variation items, this step further includes:
[0053] Step 2.1, using the nuclear norm to represent the low-rank pure hyperspectral image f 1 (L)=||L|| * , where L is a low-rank matrix, repr...
PUM
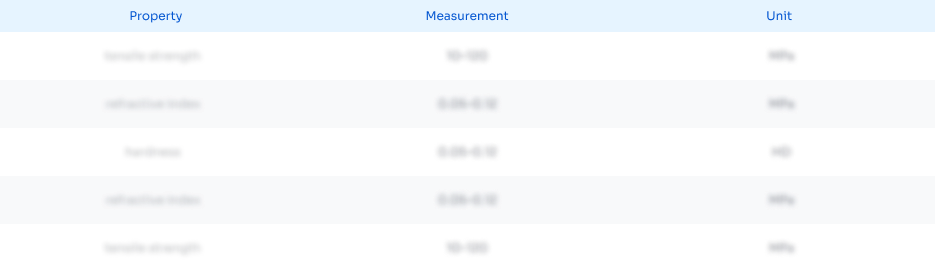
Abstract
Description
Claims
Application Information
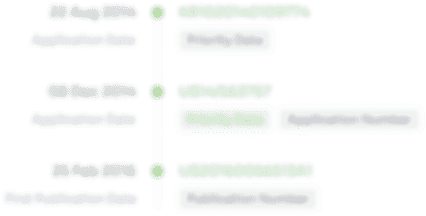
- R&D
- Intellectual Property
- Life Sciences
- Materials
- Tech Scout
- Unparalleled Data Quality
- Higher Quality Content
- 60% Fewer Hallucinations
Browse by: Latest US Patents, China's latest patents, Technical Efficacy Thesaurus, Application Domain, Technology Topic, Popular Technical Reports.
© 2025 PatSnap. All rights reserved.Legal|Privacy policy|Modern Slavery Act Transparency Statement|Sitemap|About US| Contact US: help@patsnap.com