Video behavior category identification method based on time domain inference graph
A recognition method and time-domain technology, applied in character and pattern recognition, instruments, biological neural network models, etc., can solve problems such as unfavorable capture action structures, and achieve the effect of improving the accuracy of category recognition
- Summary
- Abstract
- Description
- Claims
- Application Information
AI Technical Summary
Problems solved by technology
Method used
Image
Examples
Embodiment 1
[0054] A video behavior category recognition method based on temporal inference graphs. According to the action dependencies between video frames, a multi-headed temporal adjacency matrix of multiple temporal inference graphs is constructed to infer the implicit relationship between actions and actions, and a semantic fusion device is constructed at the same time. The time-domain features of actions with different dependencies are extracted at multiple time scales and fused into a semantic feature with strong semantics for video action category recognition.
[0055] A basic behavior has both long-range and short-range dependencies, and often in a video frame-to-frame dependencies can abstract multiple relationships, e.g. consider a video of a human behavior "throwing a ball into the air, then catching it" , this behavior has many short-range and long-range basic dependencies. First, there are short-range relationships "throw", "throw into the air", "drop", "catch"; there are also...
Embodiment 2
[0061] like figure 2 As shown, a video behavior category recognition method based on time-domain inference graph, specifically includes the following steps:
[0062] Step S1: video is sampled;
[0063] Step S2: Utilize the convolutional network to extract the spatial feature X of the video frame sequence;
[0064] Step S3: construct the multi-head temporal domain adjacency matrix A that action dependency is arranged;
[0065] Step S4: use time-domain graph convolution network to reason;
[0066] Step S5: supervised training is carried out to the entire network;
[0067] Step S6: Carry out test classification to video.
Embodiment 3
[0069] like Figure 1 to Figure 4 As shown, a video behavior category recognition method based on temporal domain inference graph includes the following steps:
[0070] Step S1: Sampling the video.
[0071] A video usually has a large number of frames. If all of them are used as input for subsequent calculations, it will take a huge computational cost, and a lot of information in it is similar and redundant, so the video needs to be sampled first.
[0072] In this implementation, there are two sampling methods: the first one is global sparse sampling if the feature map is extracted by a 2D convolutional network; the second one is local dense sampling if the feature map is extracted by a 3D convolutional network.
[0073] Step S2: Use the convolutional network to extract the spatial feature X of the video frame sequence.
[0074] For the sampled video frames, use a convolutional network for feature extraction, such as 2D Inception or ResNet-50 based on 3D expansion technology...
PUM
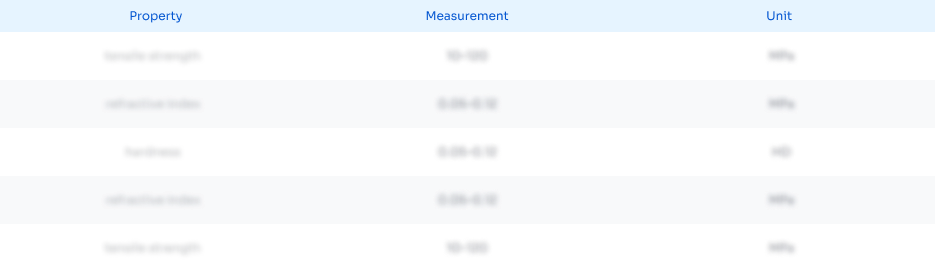
Abstract
Description
Claims
Application Information
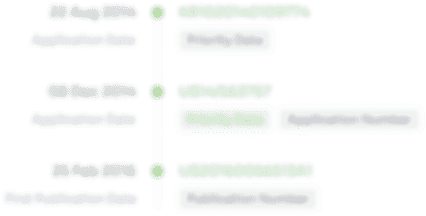
- R&D Engineer
- R&D Manager
- IP Professional
- Industry Leading Data Capabilities
- Powerful AI technology
- Patent DNA Extraction
Browse by: Latest US Patents, China's latest patents, Technical Efficacy Thesaurus, Application Domain, Technology Topic, Popular Technical Reports.
© 2024 PatSnap. All rights reserved.Legal|Privacy policy|Modern Slavery Act Transparency Statement|Sitemap|About US| Contact US: help@patsnap.com