Weak supervision fine-grained image classification algorithm based on correlation-guided discriminant learning
A classification algorithm and correlation technology, applied in the field of computer vision, can solve problems such as ignoring internal spatial correlation
- Summary
- Abstract
- Description
- Claims
- Application Information
AI Technical Summary
Problems solved by technology
Method used
Image
Examples
Embodiment Construction
[0071] In order to make the purpose, technical solution and advantages of the present invention clearer, the specific implementation manners of the present invention will be further described in detail below.
[0072] Datasets: Experimental evaluations are conducted on two benchmark datasets: Caltech-UCSD Birds-200-2011 and Stanford Cars, which are widely used competition datasets for fine-grained image classification. The CUB-200-2011 dataset covers 200 bird species and contains 11788 bird images, which are divided into a training set of 5994 images and a test set of 5794 images. The Stanford car dataset contains 16,185 images of 196 categories with about 50 groupings in each category.
[0073] Implementation Details: In all our experiments, all images are resized to 448×448. We use the fully convolutional network ResNet-50 as the feature extractor and apply "batch normalization" as the regularizer. Our optimizer uses Momentum SGD with an initial learning rate of 0.001 and ...
PUM
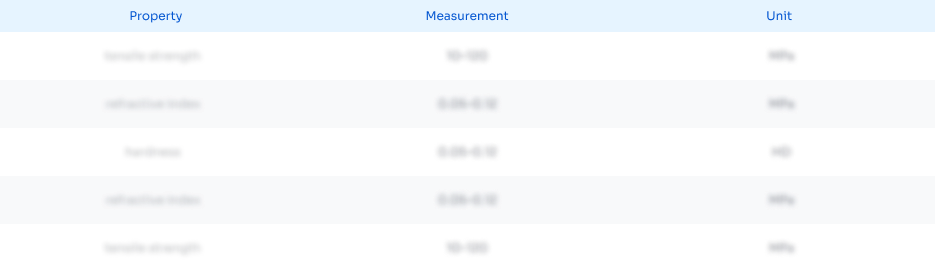
Abstract
Description
Claims
Application Information
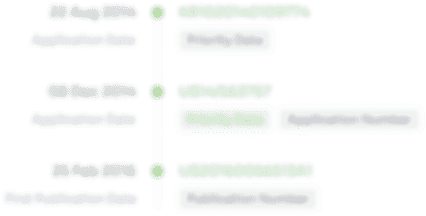
- R&D
- Intellectual Property
- Life Sciences
- Materials
- Tech Scout
- Unparalleled Data Quality
- Higher Quality Content
- 60% Fewer Hallucinations
Browse by: Latest US Patents, China's latest patents, Technical Efficacy Thesaurus, Application Domain, Technology Topic, Popular Technical Reports.
© 2025 PatSnap. All rights reserved.Legal|Privacy policy|Modern Slavery Act Transparency Statement|Sitemap|About US| Contact US: help@patsnap.com