Spatial autocorrelation machine learning satellite precipitation data downscaling method and system
A technology of spatial autocorrelation and machine learning, applied in machine learning, image data processing, nonlinear system models, etc. poor starting performance
- Summary
- Abstract
- Description
- Claims
- Application Information
AI Technical Summary
Problems solved by technology
Method used
Image
Examples
Embodiment Construction
[0087] The following is a detailed description of the embodiments of the present invention: this embodiment is implemented on the premise of the technical solution of the present invention, and provides detailed implementation methods and specific operation processes. It should be noted that those skilled in the art can make several modifications and improvements without departing from the concept of the present invention, and these all belong to the protection scope of the present invention.
[0088] The embodiment of the present invention provides a machine learning downscaling method for satellite remote sensing precipitation data considering spatial autocorrelation. First, perform spatial autocorrelation analysis on 25km TRMM monthly precipitation data, calculate the spatial autocorrelation value, and use surface-to-point Kerry The gold interpolation method is interpolated to obtain the spatial autocorrelation value of 1km precipitation data; the 1km NDVI, DEM, daytime surf...
PUM
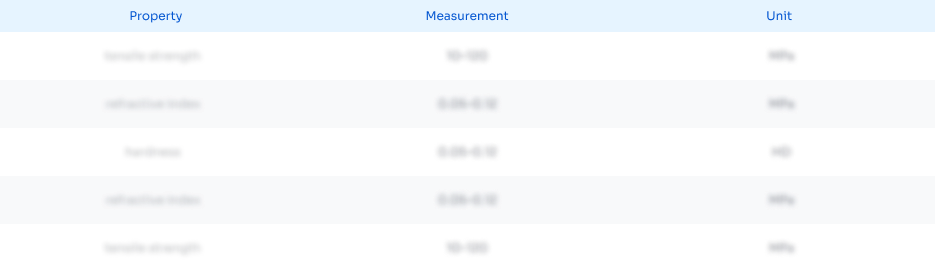
Abstract
Description
Claims
Application Information
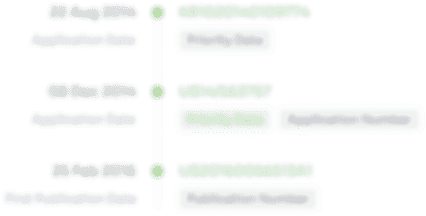
- R&D Engineer
- R&D Manager
- IP Professional
- Industry Leading Data Capabilities
- Powerful AI technology
- Patent DNA Extraction
Browse by: Latest US Patents, China's latest patents, Technical Efficacy Thesaurus, Application Domain, Technology Topic, Popular Technical Reports.
© 2024 PatSnap. All rights reserved.Legal|Privacy policy|Modern Slavery Act Transparency Statement|Sitemap|About US| Contact US: help@patsnap.com