Self-learning-based variable working condition mechanical fault intelligent diagnosis method under small sample
A technology of mechanical failure and intelligent diagnosis, applied in the direction of neural learning methods, computer components, instruments, etc., can solve problems such as difficulty in effective feature extraction, improve the ability to deal with variable working conditions, improve accuracy, and enhance training The effect of the dataset
- Summary
- Abstract
- Description
- Claims
- Application Information
AI Technical Summary
Problems solved by technology
Method used
Image
Examples
Embodiment Construction
[0048] Below in conjunction with accompanying drawing, the present invention is described in further detail:
[0049] see figure 1 , in order to improve the accuracy of fault diagnosis of mechanical equipment under variable working conditions under small samples, the present invention provides a self-learning based intelligent fault diagnosis method for mechanical equipment under variable working conditions under small samples, including the following steps:
[0050] Step 1: Take mechanical signal samples under variable operating conditions (variable load, variable speed, noise interference) of mechanical equipment as the data set, and the number of mechanical signal samples in each operating state shall not exceed 30. Signal samples are preprocessed for normalization.
[0051] The data standardization preprocessing uses zero-mean normalization, and the calculation formula is:
[0052]
[0053]
[0054]
[0055] In the formula, n is the number of data points of a si...
PUM
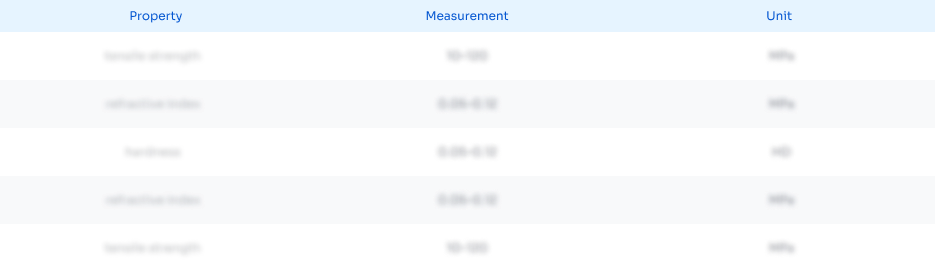
Abstract
Description
Claims
Application Information
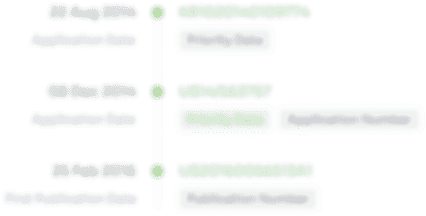
- R&D Engineer
- R&D Manager
- IP Professional
- Industry Leading Data Capabilities
- Powerful AI technology
- Patent DNA Extraction
Browse by: Latest US Patents, China's latest patents, Technical Efficacy Thesaurus, Application Domain, Technology Topic, Popular Technical Reports.
© 2024 PatSnap. All rights reserved.Legal|Privacy policy|Modern Slavery Act Transparency Statement|Sitemap|About US| Contact US: help@patsnap.com