A Transformer Fault Diagnosis Method Based on Particle Swarm Optimization Optimization Random Forest Model
A random forest model, transformer fault technology, applied in computational models, biological models, instruments, etc., can solve the problems of absolute fault boundary distinction, difficulty in obtaining the global optimal solution, long BPNN training time, etc., to improve the accuracy rate Effect
- Summary
- Abstract
- Description
- Claims
- Application Information
AI Technical Summary
Problems solved by technology
Method used
Image
Examples
Embodiment
[0078] Collect the dissolved gas sample data of known faulty transformers, and use all the collected data samples to form a total of 1723 groups of transformer fault data sets, which are divided into 1378 groups of training set data samples and 345 groups of test set data samples according to 8:2. On this basis, the analysis is carried out to verify the performance of the particle swarm optimization algorithm to optimize the random forest model. The samples of each fault type are divided in proportion as shown in Table 1.
[0079] Table 1 Data distribution of failure samples
[0080]
[0081]
[0082] According to the data division in Table 1, the uncoded ratio of the dissolved gas in the transformer oil is input as a characteristic parameter into the particle swarm optimization random forest (PSO-RF) model to optimize two key parameters, the number of subtrees n_trees and the number of split features m_features, Particle fitness is obtained from the diagnostic accuracy ...
PUM
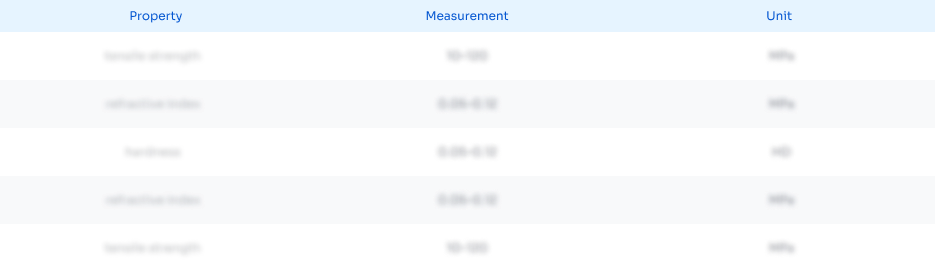
Abstract
Description
Claims
Application Information
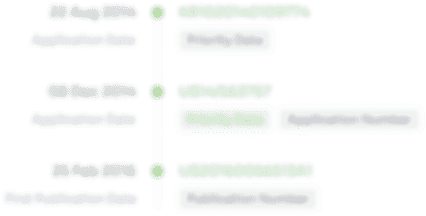
- R&D Engineer
- R&D Manager
- IP Professional
- Industry Leading Data Capabilities
- Powerful AI technology
- Patent DNA Extraction
Browse by: Latest US Patents, China's latest patents, Technical Efficacy Thesaurus, Application Domain, Technology Topic.
© 2024 PatSnap. All rights reserved.Legal|Privacy policy|Modern Slavery Act Transparency Statement|Sitemap