Image classification method for two-way channel attention element learning
A technology of two-way channel and classification method, which is applied in the field of image classification of two-way channel attention meta-learning, which can solve the problems of insufficient attention to detail areas, ignorance, and significant image features.
- Summary
- Abstract
- Description
- Claims
- Application Information
AI Technical Summary
Problems solved by technology
Method used
Image
Examples
Embodiment Construction
[0029] An image classification method for two-way channel attention meta-learning of the present invention will be described in detail below with reference to the embodiments and the accompanying drawings.
[0030] like figure 1 Shown, the image classification method of a kind of two-way channel attention element learning of the present invention, comprises the following steps:
[0031] 1) Divide the image data into Meta Train Set and Meta Test Set; Divide Meta Training Set and Meta Test Set into Support Set and Query Set respectively, Take a set amount of samples for each category in the support set to form a few-sample task (episodes);
[0032] 2) Randomly obtain C image categories from the support set of the meta-training set, and each image category contains K image visual features and recorded as: x S ={x 1 ,x 2 ,x 3 ,...,x N}, where N=K×C, is the number of images in the support set; the image visual feature x is obtained from the query set of the meta-training set ...
PUM
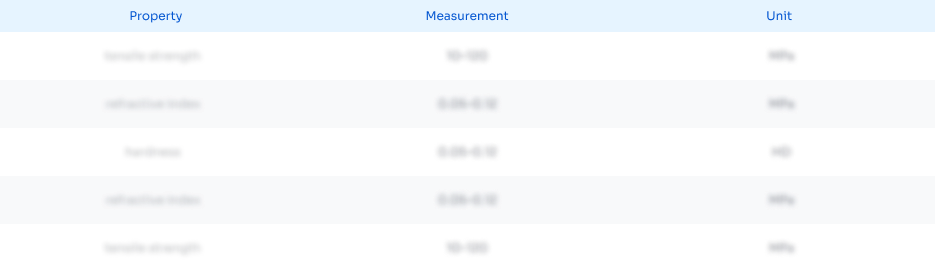
Abstract
Description
Claims
Application Information
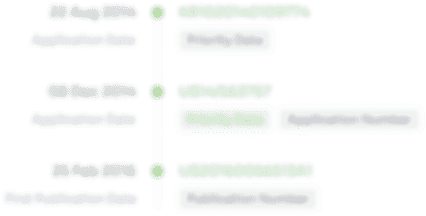
- R&D Engineer
- R&D Manager
- IP Professional
- Industry Leading Data Capabilities
- Powerful AI technology
- Patent DNA Extraction
Browse by: Latest US Patents, China's latest patents, Technical Efficacy Thesaurus, Application Domain, Technology Topic, Popular Technical Reports.
© 2024 PatSnap. All rights reserved.Legal|Privacy policy|Modern Slavery Act Transparency Statement|Sitemap|About US| Contact US: help@patsnap.com