Fan multi-fault diagnosis method based on deep metric network difficult for sample mining
A diagnostic method and multi-fault technology, applied in neural learning methods, biological neural network models, computer components, etc., can solve problems such as poor performance and limited number of fault types
- Summary
- Abstract
- Description
- Claims
- Application Information
AI Technical Summary
Problems solved by technology
Method used
Image
Examples
Embodiment Construction
[0047] The specific implementation manners of the present invention will be further described in detail below in conjunction with the accompanying drawings and embodiments. The following examples are used to illustrate the present invention, but are not intended to limit the scope of the present invention.
[0048] Multi-fault diagnosis method for wind turbines based on deep metric network of hard sample mining, such as figure 1 shown, including the following steps:
[0049] Step 1: Build a difficult training sample mining model; for a wind turbine z different types of SCADA (Supervisory Control And Data Acquisition, that is, data acquisition and monitoring control system data) data sets, D = [D 1 ,D 2 ,...,D z ] T , where each type includes normal data R n , abnormal data R f ; In order to select a sample set with high training accuracy, the abnormal data R f Divided into early failure data S ef and fault data S f , sample equalization is performed on normal data and...
PUM
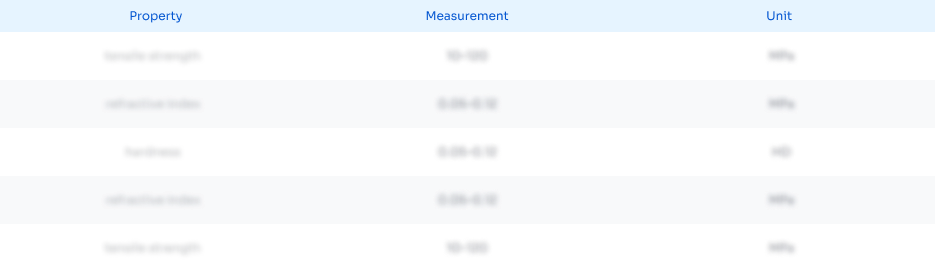
Abstract
Description
Claims
Application Information
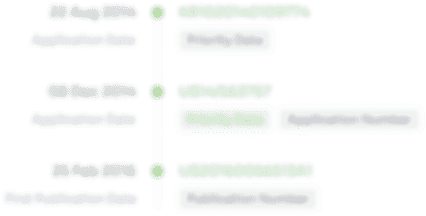
- R&D Engineer
- R&D Manager
- IP Professional
- Industry Leading Data Capabilities
- Powerful AI technology
- Patent DNA Extraction
Browse by: Latest US Patents, China's latest patents, Technical Efficacy Thesaurus, Application Domain, Technology Topic.
© 2024 PatSnap. All rights reserved.Legal|Privacy policy|Modern Slavery Act Transparency Statement|Sitemap