An Indoor Positioning Method Based on Deep Adaptive Network
An adaptive network and indoor positioning technology, which is applied in the field of indoor positioning based on a deep adaptive network, can solve problems such as difficult to form accurate, real-time, and stable positioning, achieve high positioning accuracy, improve accuracy and robustness, and be robust good sex effect
- Summary
- Abstract
- Description
- Claims
- Application Information
AI Technical Summary
Problems solved by technology
Method used
Image
Examples
Embodiment 1
[0050] A Deep Adaptive Network Based Indoor Localization Method.
[0051] A kind of indoor localization method based on deep adaptive network, it is characterized in that comprising the following steps:
[0052] Step 1. Divide the indoor environment to be positioned into grid areas of equal size, and record the corresponding coordinate information;
[0053] Step 2. Place the mobile device at each grid point in the positioning environment in turn, and record the RSS value and the corresponding grid point coordinate information from each access point at the moment to form an RSS offline fingerprint database, which is the source domain;
[0054] Step 3. collect the RSS value of the mobile equipment to be located, form the target field;
[0055] Step 4. Knowledge transfer;
[0056] Step 5. Input the target domain data into the trained network to obtain the position.
Embodiment 2
[0058] A kind of indoor localization method based on deep adaptive network, it is characterized in that comprising the following steps:
[0059] Step 1. Divide the indoor environment to be positioned into grid areas of equal size, and record the corresponding coordinate information;
[0060] Step 2. Place the mobile device at each grid point in the positioning environment in turn, and record the RSS value and the corresponding grid point coordinate information from each access point at the moment to form an RSS offline fingerprint database, which is the source domain;
[0061] Step 3. collect the RSS value of the mobile equipment to be located, form the target field;
[0062] Step 4. Knowledge transfer;
[0063] Step 5. Input the target domain data into the trained network to obtain the position.
[0064] The detailed steps of step 2 are as follows: acquire data and form an RSS fingerprint library, place the mobile device in each grid point in turn, record the grid point num...
Embodiment 3
[0074] A kind of indoor localization method based on deep adaptive network, it is characterized in that comprising the following steps:
[0075] Step 1. Divide the indoor environment to be positioned into grid areas of equal size, and record the corresponding coordinate information;
[0076] Step 2. Place the mobile device at each grid point in the positioning environment in turn, and record the RSS value and the corresponding grid point coordinate information from each access point at the moment to form an RSS offline fingerprint database, which is the source domain;
[0077] Step 3. collect the RSS value of the mobile equipment to be located, form the target field;
[0078] Step 4. Knowledge transfer;
[0079] Step 5. Input the target domain data into the trained network to obtain the position.
[0080] The detailed steps of step 2 are as follows: acquire data and form an RSS fingerprint library, place the mobile device in each grid point in turn, record the grid point num...
PUM
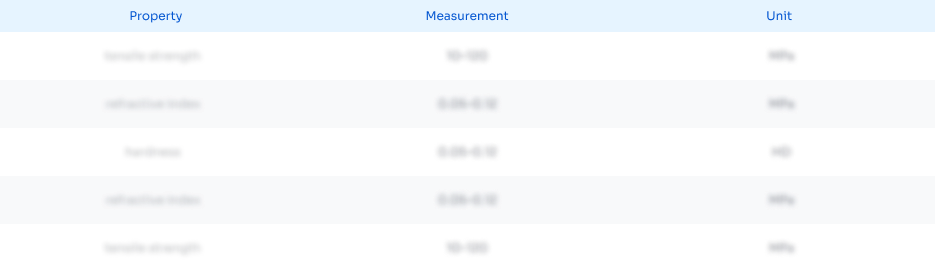
Abstract
Description
Claims
Application Information
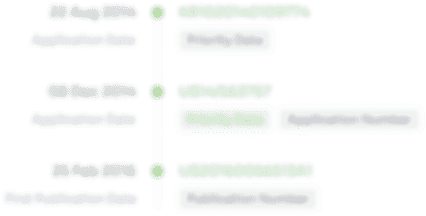
- R&D Engineer
- R&D Manager
- IP Professional
- Industry Leading Data Capabilities
- Powerful AI technology
- Patent DNA Extraction
Browse by: Latest US Patents, China's latest patents, Technical Efficacy Thesaurus, Application Domain, Technology Topic, Popular Technical Reports.
© 2024 PatSnap. All rights reserved.Legal|Privacy policy|Modern Slavery Act Transparency Statement|Sitemap|About US| Contact US: help@patsnap.com