Millimeter wave MIMO hybrid beam forming optimization method based on deep learning
An optimization method and deep learning technology, applied in space transmission diversity, radio transmission system, electrical components, etc., can solve problems such as changing priorities
- Summary
- Abstract
- Description
- Claims
- Application Information
AI Technical Summary
Problems solved by technology
Method used
Image
Examples
Embodiment 1
[0041] According to Figure 1 ~ Figure 4 , Give the preferred embodiment of the present invention, and give a certain description to enable a better understanding of the function and characteristics of the present invention.
[0042] Such as figure 1 : A complete multi-user millimeter wave massive MIMO hybrid beamforming system includes: a transmitting end, multiple receiving ends, where the transmitting end includes a baseband beamformer, a radio frequency beamformer, and multiple transmitting antennas, which are sequentially connected, and the receiving end It includes multiple receiver antennas, radio frequency combiners and baseband combiners connected in sequence. In the present invention, the same system is constructed in the manner of neural network, and the whole system is a single-cell multi-user model. will figure 1 The system shown is mapped to figure 2 Neural network in figure 2 The neural network is a cascaded neural network, which is divided into baseband beamf...
PUM
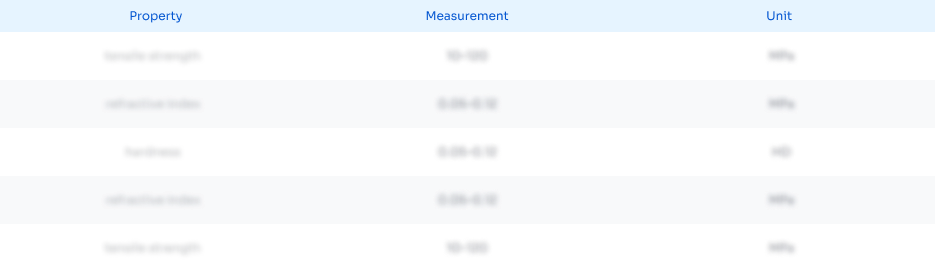
Abstract
Description
Claims
Application Information
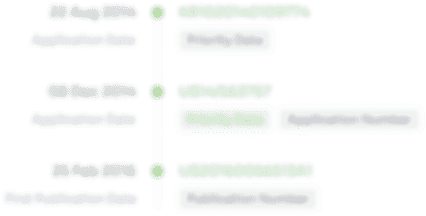
- R&D Engineer
- R&D Manager
- IP Professional
- Industry Leading Data Capabilities
- Powerful AI technology
- Patent DNA Extraction
Browse by: Latest US Patents, China's latest patents, Technical Efficacy Thesaurus, Application Domain, Technology Topic, Popular Technical Reports.
© 2024 PatSnap. All rights reserved.Legal|Privacy policy|Modern Slavery Act Transparency Statement|Sitemap|About US| Contact US: help@patsnap.com