Hyperspectral and Panchromatic Image Fusion Method Based on Spatial Features Extracted by Aae
A hyperspectral image and panchromatic image technology, applied in image enhancement, image analysis, graphic image conversion, etc., can solve the problems of sub-optimal, imperfect spectral information, and high algorithm complexity
- Summary
- Abstract
- Description
- Claims
- Application Information
AI Technical Summary
Problems solved by technology
Method used
Image
Examples
Embodiment 1
[0040] Due to the limitation of incident light energy, there is a certain balance between the spectral resolution, spatial resolution and signal-to-noise ratio of remote sensing data obtained by different sensors. Hyperspectral imaging sensors can acquire hyperspectral images with hundreds of narrow spectral channels and have high spectral resolution. Since hyperspectral images have detailed spectral information, they have unique advantages in classification, detection, and identification. However, the spatial resolution of hyperspectral images is insufficient. When processing and utilizing images, it is necessary to obtain hyperspectral images with both high spatial and spectral resolutions.
[0041] Using neural networks for image fusion is usually to learn the mapping relationship between input images and reconstructed fusion images. It has great advantages in feature extraction, data representation and description of complex relationships, but the design of neural network ...
Embodiment 2
[0076] The hyperspectral and panchromatic image fusion method based on extracting spatial features against an autoencoder is the same as in Embodiment 1, and the training process of the network described in step 3 of the present invention includes the following steps:
[0077] The adversarial autoencoder can be divided into two major network structures: the autoencoder part and the discriminative part of the generative adversarial network (GAN). After initializing the weights and biases of the adversarial autoencoder, training the adversarial autoencoder is divided into two processes:
[0078] (3.1) Reconstruction of input samples: the upsampled hyperspectral image H is used as the input of the adversarial autoencoder for training, and the loss function of the autoencoder is calculated; the stochastic gradient descent algorithm is used for optimization, and the optimization process is to minimize the loss function The process of optimizing, while updating the network weights a...
Embodiment 3
[0082] The hyperspectral and panchromatic image fusion method based on extracting spatial features against the self-encoder is the same as that in Embodiment 1-2, and the panchromatic image is enhanced as described in step 4, specifically including the following steps:
[0083] (4.1) Perform adaptive histogram equalization on the panchromatic image P:
[0084] P h =adapt thisteq(P)
[0085] Among them, P represents a panchromatic image, adaptthisteq( ) represents an adaptive histogram equalization function, and P h Represents a panchromatic image after adaptive histogram equalization;
[0086] (4.2) Use the Laplacian-Gaussian (LOG) enhancement algorithm to increase the panchromatic image P after adaptive histogram equalization h space details.
[0087] (4.2.1) Use the Gaussian filter to remove the noise of the panchromatic image after adaptive histogram equalization:
[0088] P g =P h × g
[0089] Among them, P h Represents the panchromatic image after adaptive histog...
PUM
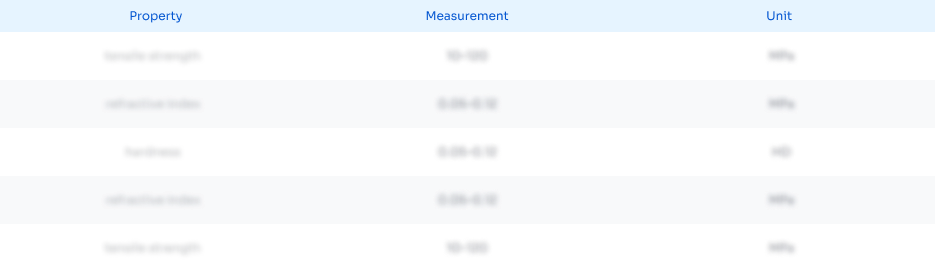
Abstract
Description
Claims
Application Information
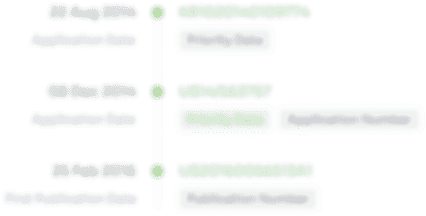
- R&D Engineer
- R&D Manager
- IP Professional
- Industry Leading Data Capabilities
- Powerful AI technology
- Patent DNA Extraction
Browse by: Latest US Patents, China's latest patents, Technical Efficacy Thesaurus, Application Domain, Technology Topic, Popular Technical Reports.
© 2024 PatSnap. All rights reserved.Legal|Privacy policy|Modern Slavery Act Transparency Statement|Sitemap|About US| Contact US: help@patsnap.com