Metallurgical enterprise energy consumption prediction method based on integrated long-term and short-term memory network
A technology that integrates long-term and short-term memory and long-term and short-term memory. It is used in prediction, neural learning methods, biological neural network models, etc., and can solve the problems of poor robustness of support vector regression prediction models.
- Summary
- Abstract
- Description
- Claims
- Application Information
AI Technical Summary
Problems solved by technology
Method used
Image
Examples
Embodiment Construction
[0042] In this embodiment, a method for predicting energy consumption of metallurgical enterprises based on integrated long-term and short-term memory network, the overall flow diagram is as follows figure 1 As shown in Figure 1, the collected energy consumption data of metallurgical enterprises is preprocessed first; then the deep learning features of energy consumption data of metallurgical enterprises are extracted by using long short-term memory network, and the training set of energy consumption data of multiple metallurgical enterprises is constructed by self-service sampling method. Train support vector regression prediction models for energy consumption data of multiple metallurgical enterprises; finally, use Jensen-Shannon divergence to select K trained support vector regression prediction models, and use adaptive linear normalization combination method to select the selected support vector regression prediction models. The results of the vector regression prediction m...
PUM
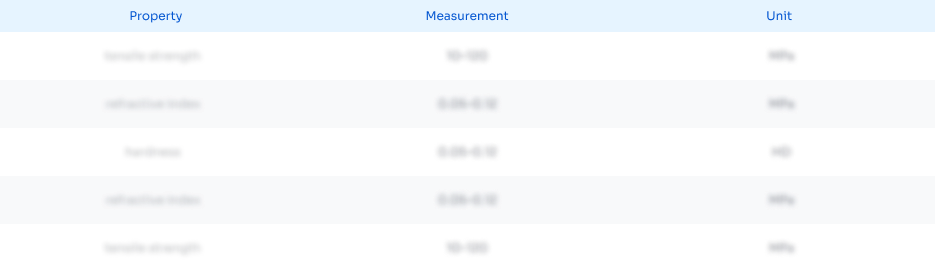
Abstract
Description
Claims
Application Information
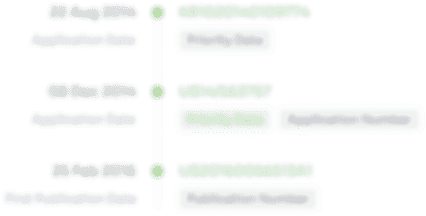
- R&D Engineer
- R&D Manager
- IP Professional
- Industry Leading Data Capabilities
- Powerful AI technology
- Patent DNA Extraction
Browse by: Latest US Patents, China's latest patents, Technical Efficacy Thesaurus, Application Domain, Technology Topic.
© 2024 PatSnap. All rights reserved.Legal|Privacy policy|Modern Slavery Act Transparency Statement|Sitemap