Semi-automatic labeling method for image data
An image data, semi-automatic technology, applied in the computer field, can solve the problems of insufficient model accuracy and poor effect, and achieve the effect of ensuring accuracy
- Summary
- Abstract
- Description
- Claims
- Application Information
AI Technical Summary
Problems solved by technology
Method used
Image
Examples
Embodiment Construction
[0021] The following examples can enable those skilled in the art to understand the present invention more fully, but do not limit the present invention in any way. The experimental methods in the following examples are conventional methods unless otherwise specified.
[0022] Before explaining the present invention, two concepts involved in the present invention are first clarified: Image-level labeling refers to labeling only its category for an image, and not labeling specific locations in each object. Instance-level annotation refers to annotating the category of an image for an image, and also specifying the specific location boxes of each target in the image.
[0023] The method of the present invention mainly includes the following steps: 1. Carry out image-level labeling to a small amount of unlabeled images; 2. Put the labeled data in figure 1 Training in the model in , this model is called the collaborative weak supervision recognition model; 3. After the training i...
PUM
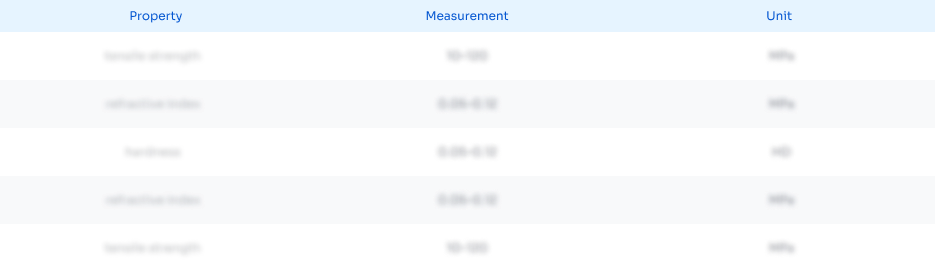
Abstract
Description
Claims
Application Information
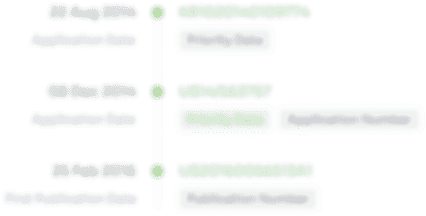
- R&D Engineer
- R&D Manager
- IP Professional
- Industry Leading Data Capabilities
- Powerful AI technology
- Patent DNA Extraction
Browse by: Latest US Patents, China's latest patents, Technical Efficacy Thesaurus, Application Domain, Technology Topic, Popular Technical Reports.
© 2024 PatSnap. All rights reserved.Legal|Privacy policy|Modern Slavery Act Transparency Statement|Sitemap|About US| Contact US: help@patsnap.com