Transaction stock rapid finding method based on time sequence convolution self-coding
A convolutional self-encoding and timing technology, applied in neural learning methods, data processing applications, finance, etc., can solve problems such as losses, investors missing trading opportunities, and reduced profits
- Summary
- Abstract
- Description
- Claims
- Application Information
AI Technical Summary
Problems solved by technology
Method used
Image
Examples
Embodiment 1
[0076] The present invention provides a method for quickly finding abnormal stocks based on time-series convolutional self-encoding, including:
[0077] S1. Obtain stock trading market data within a time span; stock trading market data such as a trading day, an economically related securities market - such as China's Shanghai and Shenzhen stock markets;
[0078] S2. Formalize the time-series data of the stock trading market data obtained in S1 to generate time-series formalized data of the stock;
[0079] S3. Generate a time-series convolutional self-encoding model according to the time-series formalized data of S2, and train the time-series convolutional self-encoding model;
[0080] S4. According to the time-series convolutional autoencoder model obtained in S3, combined with the time-series formalized data of S2, find abnormal stocks. Abnormal stocks refer to a small number of stocks that are significantly different from the trend of most stocks.
[0081] In S2, the forma...
Embodiment 2
[0111] see figure 1 , on the basis of Embodiment 1, a system for quickly finding abnormal stocks based on time-series convolutional self-encoding is proposed, including a transaction data acquisition module, a time-series data formal representation module, a model generation module, and an abnormal stock detection module;
[0112] The data input terminal of the transaction data acquisition module is connected to the stock database, and the transaction data acquisition module, the time series data formal representation module, and the transaction stock detection module are connected in sequence to form a data link;
[0113] Wherein the data output end of the transaction data acquisition module is connected to the data input end of the time series data formal representation module; the data output end of the time series data formal representation module is respectively connected to the input ends of the mobile stock detection module and the model generation module;
[0114] The ...
Embodiment 3
[0116] On the basis of Embodiment 2, a method for quickly finding abnormal stocks based on the time-series convolutional self-encoding system for quickly finding abnormal stocks is proposed,
[0117] Step 1, the transaction data acquisition module is responsible for obtaining all stock transaction data within a time span - such as a trading day, an economically related securities market - such as China's Shanghai and Shenzhen stock markets; after obtaining the data, it is imported in the form of time series data representation module;
[0118] Step 2, the time series data formal representation module is responsible for the formal representation of the incoming transaction data;
[0119] Step 3, the model generation module generates a time-series convolutional self-encoding model according to the time-series formalized data in step 2, and trains the time-series convolutional self-encoding model;
[0120] Step 4: The abnormal stock detection module finds stocks with abnormal tr...
PUM
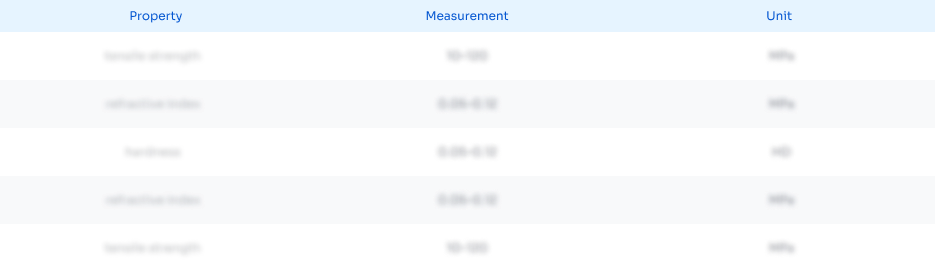
Abstract
Description
Claims
Application Information
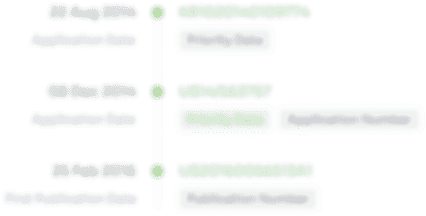
- R&D Engineer
- R&D Manager
- IP Professional
- Industry Leading Data Capabilities
- Powerful AI technology
- Patent DNA Extraction
Browse by: Latest US Patents, China's latest patents, Technical Efficacy Thesaurus, Application Domain, Technology Topic.
© 2024 PatSnap. All rights reserved.Legal|Privacy policy|Modern Slavery Act Transparency Statement|Sitemap