Factory production state abnormity detection model design method
An anomaly detection and model design technology, applied in the field of detection, can solve problems such as difficult to meet the rapid changes in the actual operation status of the factory, and achieve the effect of convenient and refined management and decision-making basis
- Summary
- Abstract
- Description
- Claims
- Application Information
AI Technical Summary
Problems solved by technology
Method used
Image
Examples
Embodiment 1
[0024] Example 1: See Figure 1-4 , a method for designing an abnormality detection model of a factory production state, comprising the following steps:
[0025] A, build support vector field; Described step A is specifically: construct sphere field, assume sphere center position a and sphere radius R, set up minimum radius function: Among them, C is a variable between the size of the coordination sphere and the error of the target object, and the constraints are: (x i -a) T (x i -a)≤R 2 +ξ i , ξ i ≥0, combine the above two formulas to create the Lagrangian function: where a i ≥0 and γ i ≥0, according to the above function, the following new constraints are obtained: C-a i -γ i =0, that is, the final Lagrangian function is as follows: By optimizing the solution to the minimization of L, it can be obtained that when the new test set data enters the model, whether it belongs to the normal category range, that is Among them, z is the new instance data.
[002...
PUM
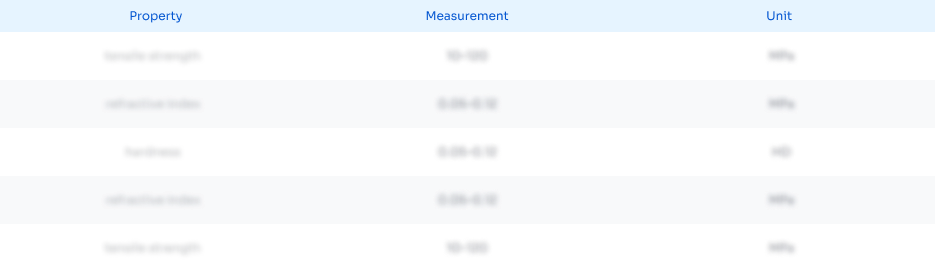
Abstract
Description
Claims
Application Information
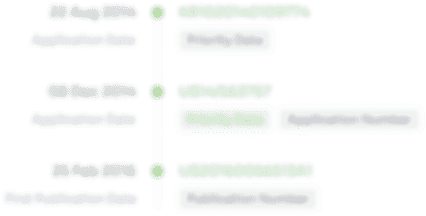
- R&D Engineer
- R&D Manager
- IP Professional
- Industry Leading Data Capabilities
- Powerful AI technology
- Patent DNA Extraction
Browse by: Latest US Patents, China's latest patents, Technical Efficacy Thesaurus, Application Domain, Technology Topic.
© 2024 PatSnap. All rights reserved.Legal|Privacy policy|Modern Slavery Act Transparency Statement|Sitemap