Semi-supervised width learning classification method based on manifold regularization and width network
A classification method, semi-supervised technology, applied in neural learning methods, biological neural network models, character and pattern recognition, etc., can solve problems such as limited applicability
- Summary
- Abstract
- Description
- Claims
- Application Information
AI Technical Summary
Problems solved by technology
Method used
Image
Examples
Embodiment 1
[0142] In Example 1, a series of experiments are carried out on the classic artificial dataset G50C. The G50C dataset is a standard dataset derived from the KEEL database for semi-supervised classification. It is a binary classification dataset in which each class is generated by a 50-D multivariate Gaussian distribution. This classification problem is explicitly designed so that the true Bayes error is about 5%. The dataset consists of 550 samples.
[0143] In order to test the accuracy and efficiency of the proposed method, in this experiment, the G50C data set is divided into 10 labeled training sample sets, 350 unlabeled training sample sets, and 100 labeled test sample sets. The structure of DBN is set to 168-64-32, the number of hidden layer nodes of SS-ELM is set to 1000, the structure of SS-HELM is set to 50-50-500, the structure of SS-BLS is set to 10-10-500, in In this experiment, the parameter selection of the three algorithms is shown in Table 1:
[0144] Table ...
Embodiment 2
[0155] In the second embodiment, a series of experiments are carried out on the classic MNIST handwritten digit images, and the data set consists of 70,000 handwritten digits. Each digit is represented by an image of size 28 × 28 grayscale pixels. Typically, its image is displayed in the Image 6 middle.
[0156] In order to test the performance of the proposed model, in this experiment, the MNIST data set used is 100 labeled training sample sets, 9000 unlabeled training sample sets, and 60000 test sample sets. For reference, the deep structure of DBN is 128-64-32, the structure of SS-HELM is set to 100-100-3000, and the number of hidden layer nodes of SS-ELM is set to 4000. In addition, the parameter selection of DBN is the same as that described in 5.1, and the parameter selection of SS-ELM, SS-BLS, and SS-HELM is shown in Table 3:
[0157] Table 3 Selection of regularization parameters for semi-supervised classifiers on the MNIST dataset
[0158] parameter S...
Embodiment 3
[0172] In the third embodiment, compared with the MNIST data set, the NORB data set is a more complex data set; each image has 2 × 32 × 32 pixels, and there are 48600 images in total. The NORB dataset contains images of 50 different 3D toy objects belonging to 5 different categories: 1) animals; 2) humans; 3) airplanes; 4) trucks; 5) cars, sampled objects are imaged under various lighting conditions and orientations ,Such as Figure 11 shown.
[0173] The training set contains 24300 images of 25 objects (5 of each class), while the test set contains 24300 images of the remaining 25 objects. In the experiment, this paper divides the data into 1000 labeled training sample sets, 14300 unlabeled training sample sets, and 24300 labeled test sample sets. For comparison, the structure of DBN is 128-64-32, SS - The structure of HELM is set to 500-500-3000, and the number of hidden layer nodes of SS-ELM is set to 5000. In addition, the parameter selection of DBN is the same as that ...
PUM
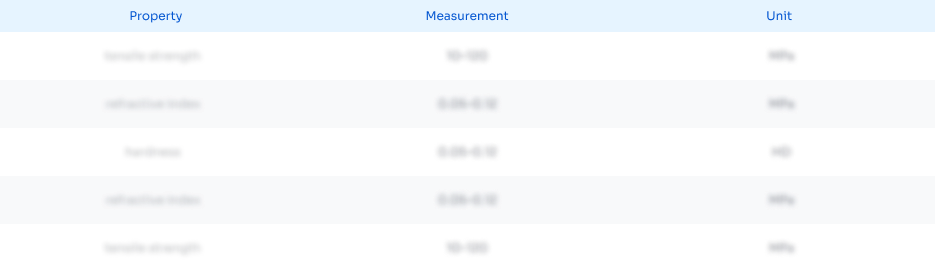
Abstract
Description
Claims
Application Information
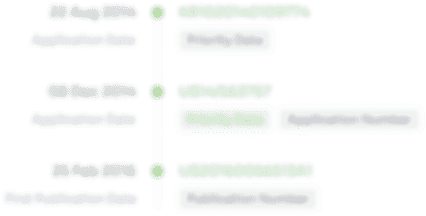
- R&D Engineer
- R&D Manager
- IP Professional
- Industry Leading Data Capabilities
- Powerful AI technology
- Patent DNA Extraction
Browse by: Latest US Patents, China's latest patents, Technical Efficacy Thesaurus, Application Domain, Technology Topic, Popular Technical Reports.
© 2024 PatSnap. All rights reserved.Legal|Privacy policy|Modern Slavery Act Transparency Statement|Sitemap|About US| Contact US: help@patsnap.com