A video deshaking method based on deep learning
A technology of video de-shaking and deep learning, applied in the field of computer vision and video de-shaking, which can solve the problems of limitations and less research on video de-shaking, and achieve the effects of increasing stability, improving precision, and ensuring rationality and accuracy
- Summary
- Abstract
- Description
- Claims
- Application Information
AI Technical Summary
Problems solved by technology
Method used
Image
Examples
Embodiment Construction
[0059] The present invention will be described in detail below in conjunction with the accompanying drawings and embodiments.
[0060] Such as figure 1 Shown, the inventive method comprises the steps:
[0061] Step1: In the training phase, the continuous shaking frame sequence is used as the input of the network, and the stable frame is used as the output of the network for supervised training to generate a weighted deep network;
[0062] Step2: In the test phase, the continuous jitter frame sequence is used as the input of the network to generate a pixel-level map;
[0063] Step3: During the test phase, the shaking frame is mapped point by point through the map generated by Step2 to generate a stable frame.
[0064] In the training step of the deep network, the continuous shaking frame sequence is used as the input of the network, and the stable frame is used as the output of the network for supervised training to generate a weighted deep network;
[0065] In the test pha...
PUM
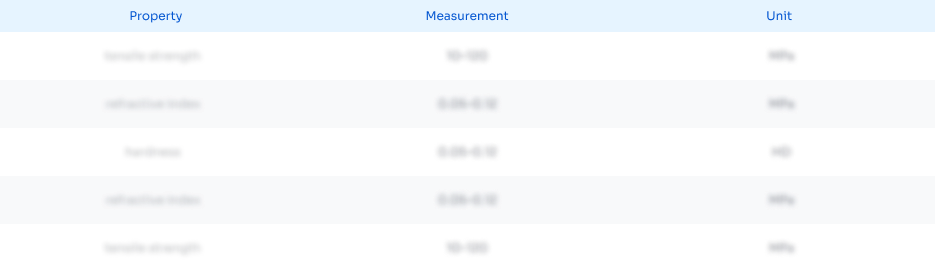
Abstract
Description
Claims
Application Information
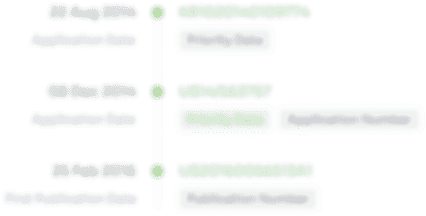
- R&D Engineer
- R&D Manager
- IP Professional
- Industry Leading Data Capabilities
- Powerful AI technology
- Patent DNA Extraction
Browse by: Latest US Patents, China's latest patents, Technical Efficacy Thesaurus, Application Domain, Technology Topic.
© 2024 PatSnap. All rights reserved.Legal|Privacy policy|Modern Slavery Act Transparency Statement|Sitemap