A method for repairing missing traffic data based on Bayesian enhanced tensor
A repair method and technology for traffic data, which are applied in directions based on specific mathematical models, traffic control systems for road vehicles, traffic flow detection, etc. Problems such as poor model interpretability, to ensure the repair effect, the repair effect is significant, and the effect of preventing overfitting
- Summary
- Abstract
- Description
- Claims
- Application Information
AI Technical Summary
Problems solved by technology
Method used
Image
Examples
Embodiment 1
[0115] Such as figure 1 As shown, a missing traffic data restoration method based on Bayesian enhanced tensor decomposition requires modeling before traffic data restoration. The specific method steps are as follows:
[0116]D1: Divide the space-time dimension, and organize the road speed data into a high-order tensor. Specifically, the road speed data is collected from floating cars, and the floating cars on the road are aggregated according to the specified time window (for example, 10 minutes, then 144 time windows are formed in one day), and the speed data sequence of each road section in the time dimension can be obtained. Considering that in the time dimension, traffic data has different modes such as day, week and month, the time dimension can be further divided. In this embodiment, in terms of time dimension, two dimensions of day and time window are extracted. Therefore, the road network speed data can be organized into a third-order tensor for each y in the tenso...
PUM
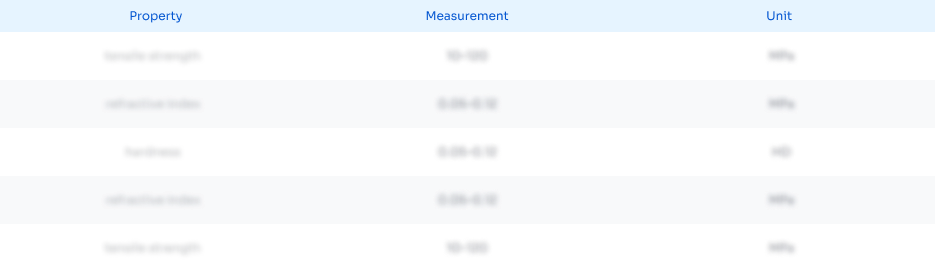
Abstract
Description
Claims
Application Information
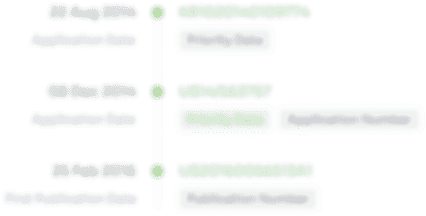
- R&D Engineer
- R&D Manager
- IP Professional
- Industry Leading Data Capabilities
- Powerful AI technology
- Patent DNA Extraction
Browse by: Latest US Patents, China's latest patents, Technical Efficacy Thesaurus, Application Domain, Technology Topic, Popular Technical Reports.
© 2024 PatSnap. All rights reserved.Legal|Privacy policy|Modern Slavery Act Transparency Statement|Sitemap|About US| Contact US: help@patsnap.com