Prediction method for retail sales volume of gas station convenience store
A forecasting method and convenience store technology, applied in marketing, commerce, instruments, etc., can solve problems such as less research work and immature theories
- Summary
- Abstract
- Description
- Claims
- Application Information
AI Technical Summary
Problems solved by technology
Method used
Image
Examples
Embodiment 1
[0086] Embodiment 1: A method for predicting retail sales of gas station convenience stores, the specific steps are as follows:
[0087] (1) Establish an unbiased gray prediction GM(1,1) model:
[0088] (1.1) First assume that the original data sequence is
[0089] x (0) =(X (0) (1),X (0) (2),...,X (0) (n)) (1)
[0090] (1.2) Then use the accumulation of the original data sequence to generate a new sequence as:
[0091] x (1) =(X (1) (1),X (1) (2),...,X (1) (n)) (2)
[0092] in,
[0093] (1.3) Calculate parameter values:
[0094] Get the parameter column by the method of least squares
[0095]
[0096] In the formula:
[0097]
[0098] (1.4) Establish an unbiased GM(1,1) prediction model:
[0099] Suppose the original data sequence satisfies:
[0100]
[0101] Where: k=1,2,...,n, a 1 ,b 1 is the undetermined coefficient;
[0102] Each x of formula (4) (0) (k) Carry out an accumulation to get:
[0103]
[0104] Substituting equations (4) and (...
Embodiment 2
[0162] Example 2: Select the sales data of CNPC Kunming Hongwa Station Convenience Store from the first quarter of 2015 to the third quarter of 2018 as a data sample, and establish an unbiased gray PSO-Markov prediction model according to the method in this paper. The parameters of PSO optimization are set as follows: the number of particle swarms m=500, the maximum number of iterations iter max = 200, particle velocity v∈[-0.1,0.1], particle position y∈[-2,2], c 1 = c 2 =2. The whitening weight function of different states obtained by improving the PSO-Markov algorithm is: a 1 =0.8030,a 2 =0.1619,a 3 = 0.0015.
[0163] The PMGM(1,1) prediction model based on PSO-Markov optimization was compared with the Markov-optimized MGM(1,1) prediction model and the unbiased gray GM(1,1) prediction model. Taking gas station convenience stores from the first quarter of 2015 to the third quarter of 2018 as the known data, the above modeling methods are used to build models to predict ...
PUM
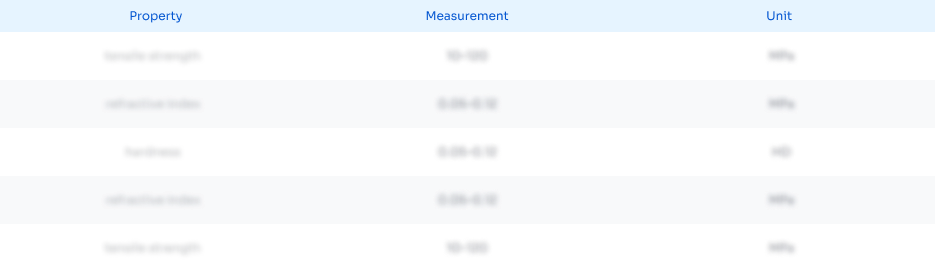
Abstract
Description
Claims
Application Information
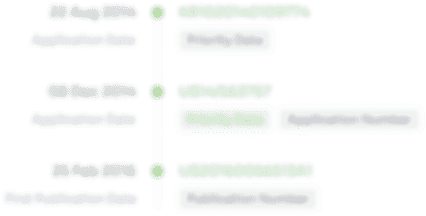
- R&D Engineer
- R&D Manager
- IP Professional
- Industry Leading Data Capabilities
- Powerful AI technology
- Patent DNA Extraction
Browse by: Latest US Patents, China's latest patents, Technical Efficacy Thesaurus, Application Domain, Technology Topic, Popular Technical Reports.
© 2024 PatSnap. All rights reserved.Legal|Privacy policy|Modern Slavery Act Transparency Statement|Sitemap|About US| Contact US: help@patsnap.com