CUDA-based Gridding algorithm optimization method and device
An optimization method and a technology for optimizing devices, which are applied in the field of parallelization, can solve problems such as low timeliness, and achieve the effects of improving operating efficiency, reducing economic costs, and saving time and cost
- Summary
- Abstract
- Description
- Claims
- Application Information
AI Technical Summary
Problems solved by technology
Method used
Image
Examples
Embodiment 1
[0040] Such as figure 1 As shown, a CUDA-based Gridding algorithm optimization method includes the following steps:
[0041] Step S101: According to the number of function calls in the Gridding algorithm, the top M functions with the highest calling times are obtained; specifically, the main functions that can be optimized in the Gridding algorithm and the number of times they are called are shown in Table 1.
[0042] Table 1 The main functions that can be optimized in the Gridding algorithm and the number of times they are called
[0043]
[0044]
[0045] It can be seen from Table 1 that the grdsf function is called the most times, reaching 370 times, anti_aliasing_calculate (anti-aliasing function) is called 185 times, convolutional_degrid (convolution degrid function) is called 47 times, convolutional_grid (convolution grid function) was called 44 times, weight_gridding was called 2 times, gridder and gridder_numba were not called. In this embodiment, the top three...
Embodiment 2
[0082] Such as Figure 5 As shown, a CUDA-based Gridding algorithm optimization device includes:
[0083] The comparison module 201 is used to obtain the top M functions with the highest number of calls according to the number of function calls in the Gridding algorithm;
[0084] The parallelization module 202 is configured to perform GPU parallelization processing on the M functions in CUDA.
[0085] Specifically, it also includes:
[0086] The replacement module 203 is configured to implement a GPU-based Gridding algorithm in CUDA, and replace the Gridding algorithm in the ARL algorithm library.
[0087] Specifically, as Figure 6 As shown, the parallelization module 202 includes:
[0088] The allocation sub-module 2021 is used to allocate an array on the video memory for the M functions that need to be parallelized by the GPU;
[0089] The transmission sub-module 2022 is used to transmit the data to be processed from the internal memory to the video memory;
[0090] D...
PUM
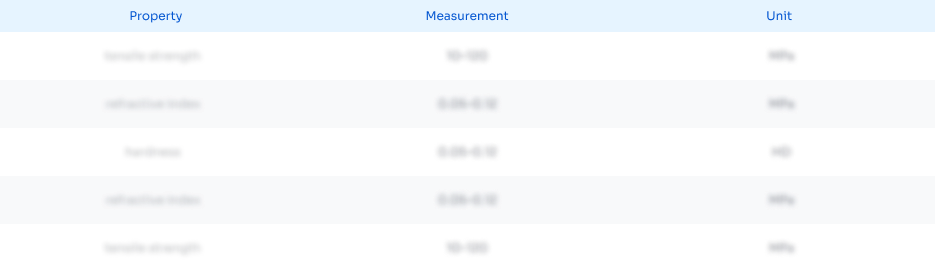
Abstract
Description
Claims
Application Information
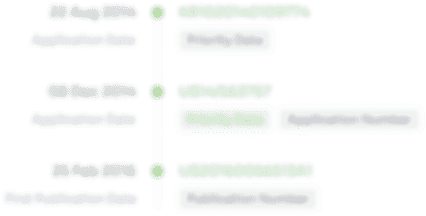
- Generate Ideas
- Intellectual Property
- Life Sciences
- Materials
- Tech Scout
- Unparalleled Data Quality
- Higher Quality Content
- 60% Fewer Hallucinations
Browse by: Latest US Patents, China's latest patents, Technical Efficacy Thesaurus, Application Domain, Technology Topic, Popular Technical Reports.
© 2025 PatSnap. All rights reserved.Legal|Privacy policy|Modern Slavery Act Transparency Statement|Sitemap|About US| Contact US: help@patsnap.com