Cross-city traffic flow joint prediction method based on deep migration learning
A technology of transfer learning and urban traffic, which is applied in the field of cross-city traffic flow joint forecasting based on deep transfer learning, can solve problems such as insufficient data in target cities, and achieve the effect of improving traffic forecasting
- Summary
- Abstract
- Description
- Claims
- Application Information
AI Technical Summary
Problems solved by technology
Method used
Image
Examples
Embodiment Construction
[0039] The technical solution of the present invention will be further described in detail below in conjunction with the accompanying drawings.
[0040] A data-sparse urban traffic flow prediction method based on deep transfer learning such as figure 1 shown. The modeled data is input into an entire network model to generate a forecast of urban traffic flow for a period of time in the future, and the input data is historical traffic flow data for training. Specifically, the present invention constructs the following data as input:
[0041] x t : The traffic flow data at n moments before the forecast time point. x t ={x t |t=1,...n}
[0042] The data-sparse city traffic flow prediction method based on deep transfer learning disclosed by the present invention has the following specific process:
[0043] Step 1: Data Preprocessing
[0044] 1) Regional division: divide the city into an m*n grid map according to latitude and longitude, and call each grid a sub-region, and a...
PUM
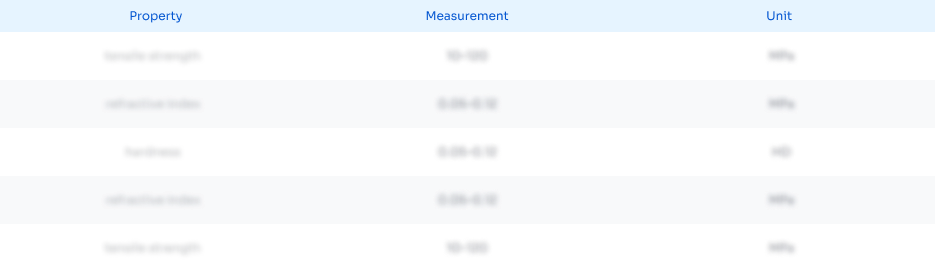
Abstract
Description
Claims
Application Information
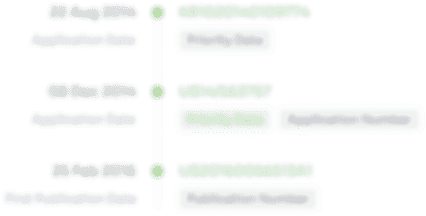
- R&D Engineer
- R&D Manager
- IP Professional
- Industry Leading Data Capabilities
- Powerful AI technology
- Patent DNA Extraction
Browse by: Latest US Patents, China's latest patents, Technical Efficacy Thesaurus, Application Domain, Technology Topic, Popular Technical Reports.
© 2024 PatSnap. All rights reserved.Legal|Privacy policy|Modern Slavery Act Transparency Statement|Sitemap|About US| Contact US: help@patsnap.com