A rolling bearing fault diagnosis method under variable working conditions based on deep features and transfer learning
A rolling bearing and transfer learning technology, which is applied in mechanical bearing testing, special data processing applications, instruments, etc., can solve problems such as low accuracy of state recognition, inability to obtain, and difficulty in labeling vibration data
- Summary
- Abstract
- Description
- Claims
- Application Information
AI Technical Summary
Problems solved by technology
Method used
Image
Examples
Embodiment Construction
[0089] This embodiment combines Figure 1 to Figure 7 , the implementation means, implementation process and performance verification of the rolling bearing fault diagnosis method under variable working conditions based on deep features and transfer learning are explained as follows:
[0090] 1 Principle of Sparse Denoising Autoencoder
[0091] 1.1 Sparse Autoencoder
[0092] Suppose h j (x) is the activation value of the hidden neuron when the input of the sparse autoencoder (Sparse Auto Encoder, SAE) is x. The average activation value of hidden neuron j can be expressed as:
[0093]
[0094] When the average activation of neurons in the hidden layer is particularly small, it can be understood as a sparsity limit, which can be expressed as Among them, ρ is a sparsity parameter, and its size is generally very close to 0. In order to satisfy the sparsity, a sparsity restriction is added to the cost function as an additional penalty factor. The specific expression is: ...
PUM
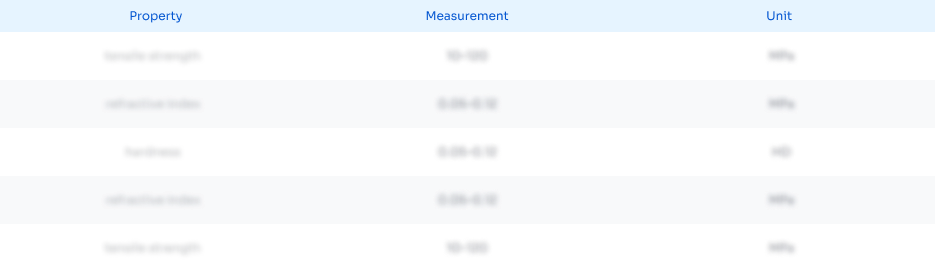
Abstract
Description
Claims
Application Information
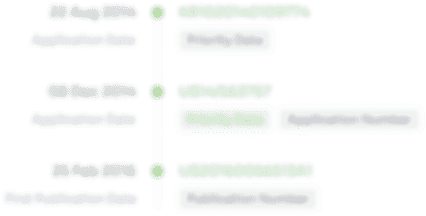
- R&D Engineer
- R&D Manager
- IP Professional
- Industry Leading Data Capabilities
- Powerful AI technology
- Patent DNA Extraction
Browse by: Latest US Patents, China's latest patents, Technical Efficacy Thesaurus, Application Domain, Technology Topic, Popular Technical Reports.
© 2024 PatSnap. All rights reserved.Legal|Privacy policy|Modern Slavery Act Transparency Statement|Sitemap|About US| Contact US: help@patsnap.com