Image super-resolution reconstruction method based on self-attention high-order fusion network
A technology of super-resolution reconstruction and network fusion, applied in the field of intelligent image processing, to achieve the effect of increasing diversity, enhancing expression ability, and solving additional calculation load
- Summary
- Abstract
- Description
- Claims
- Application Information
AI Technical Summary
Problems solved by technology
Method used
Image
Examples
Embodiment
[0041] refer to figure 1 , an image super-resolution reconstruction method based on a high-order fusion network of self-attention, including the following steps:
[0042] 1) Establish a reconstruction model: the reconstruction model includes a serial convolutional neural network and a self-attention module, such as figure 2 As shown, the convolutional neural network is equipped with a residual unit and a deconvolution layer, and the self-attention module includes a parallel attention branch and a backbone branch, and the output of the attention branch and the backbone branch is fused as a feature High-level fusion, which generates high-resolution images from low-resolution images through reconstruction models;
[0043] 2) CNN network feature extraction: directly use the original low-resolution image as the input of the CNN network established in step 1), and the output of the CNN network is a coarse-precision high-resolution feature;
[0044] 3) Feature extraction of the se...
PUM
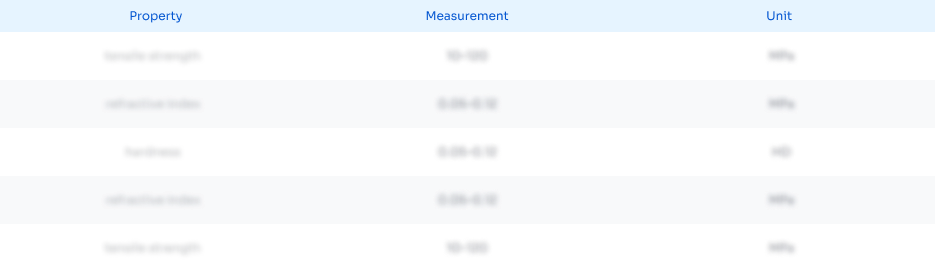
Abstract
Description
Claims
Application Information
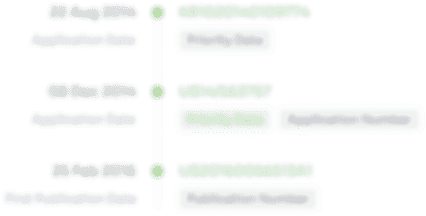
- R&D Engineer
- R&D Manager
- IP Professional
- Industry Leading Data Capabilities
- Powerful AI technology
- Patent DNA Extraction
Browse by: Latest US Patents, China's latest patents, Technical Efficacy Thesaurus, Application Domain, Technology Topic, Popular Technical Reports.
© 2024 PatSnap. All rights reserved.Legal|Privacy policy|Modern Slavery Act Transparency Statement|Sitemap|About US| Contact US: help@patsnap.com