A super-resolution image reconstruction method of a generative adversarial network based on an attention mechanism
A technology for high-resolution images and low-resolution images, which is applied in the field of super-resolution image reconstruction based on generative confrontation networks, can solve the problems of insufficient edge detail information of reconstructed images, waste of computing resources, and poor image performance, etc., to achieve The image edge and detail information are clear, the reconstruction effect is excellent, and the reconstruction effect is better
- Summary
- Abstract
- Description
- Claims
- Application Information
AI Technical Summary
Problems solved by technology
Method used
Image
Examples
specific Embodiment approach
[0036] Such as figure 1 , 2 , 3, an image super-resolution image reconstruction method based on an attention mechanism-based generation confrontation network, including the following steps:
[0037] A. Preprocess the ImageNet data set to make training data sets corresponding to high and low resolution images;
[0038] B. Construct a generative confrontation network model for training, and introduce an attention mechanism into the model;
[0039] C. Input the training data set obtained in step A into the generation confrontation network in turn for model training;
[0040] D. Input the image to be processed into the trained generation network model to obtain a reconstructed high-resolution image.
[0041] Further, the preparation method of the training data set described in step A is:
[0042] A1. Obtain the ImageNet data set, and randomly select some images as the training data set;
[0043] A2. Perform normalization processing on all the images in the obtained training d...
PUM
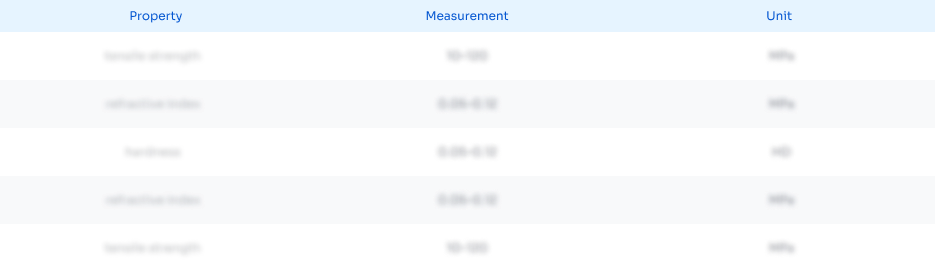
Abstract
Description
Claims
Application Information
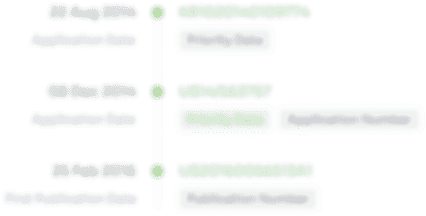
- Generate Ideas
- Intellectual Property
- Life Sciences
- Materials
- Tech Scout
- Unparalleled Data Quality
- Higher Quality Content
- 60% Fewer Hallucinations
Browse by: Latest US Patents, China's latest patents, Technical Efficacy Thesaurus, Application Domain, Technology Topic, Popular Technical Reports.
© 2025 PatSnap. All rights reserved.Legal|Privacy policy|Modern Slavery Act Transparency Statement|Sitemap|About US| Contact US: help@patsnap.com