A cross-modal hash learning method based on anchor graph
A learning method and cross-modal technology, applied in other database retrieval based on metadata, character and pattern recognition, instruments, etc., can solve the problem of keeping beneficial information of feature data and not completely solving it
- Summary
- Abstract
- Description
- Claims
- Application Information
AI Technical Summary
Problems solved by technology
Method used
Image
Examples
Embodiment Construction
[0031] A cross-modal hash learning method based on anchor graph, establishing the characteristics of n objects in image modality and text modality, respectively: and in, and Represent the feature vector of the i-th object in image mode and text mode, i=1,2,...,n,d 1 and d 2 Represent the dimensions of the feature vectors of the image modality and text modality respectively; at the same time, it is assumed that the feature vectors of the image modality and the text modality are all preprocessed by zero-centering, that is, it satisfies Assumption and are the adjacency matrices of image modality and text modality samples, respectively; matrix A (1) elements in and matrix A (2) elements in represent the similarity between the ith sample and the jth sample in the image modality and text modality, respectively; suppose S ∈ {0,1} n×n is the semantic correlation matrix between samples in the two modalities, where S ij Represents the semantic correlation between the...
PUM
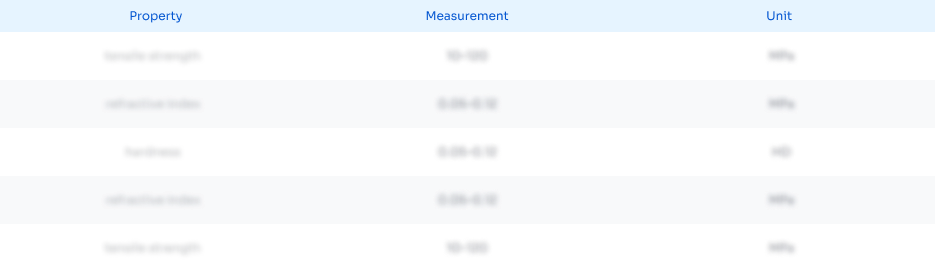
Abstract
Description
Claims
Application Information
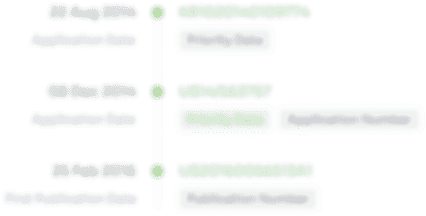
- R&D Engineer
- R&D Manager
- IP Professional
- Industry Leading Data Capabilities
- Powerful AI technology
- Patent DNA Extraction
Browse by: Latest US Patents, China's latest patents, Technical Efficacy Thesaurus, Application Domain, Technology Topic, Popular Technical Reports.
© 2024 PatSnap. All rights reserved.Legal|Privacy policy|Modern Slavery Act Transparency Statement|Sitemap|About US| Contact US: help@patsnap.com