Electricity stealing risk prediction method and device based on deep learning
A technology of deep learning and electricity stealing, applied in the field of electricity stealing risk prediction based on deep learning, can solve the problem of low accuracy of prediction results
- Summary
- Abstract
- Description
- Claims
- Application Information
AI Technical Summary
Problems solved by technology
Method used
Image
Examples
Embodiment 1
[0088] This embodiment provides a method for predicting the risk of stealing electricity based on deep learning, please refer to figure 1 , the method includes:
[0089] First, step S1 is performed: obtaining historical data from the actual application scene of the on-site metering equipment, and performing preprocessing on the obtained historical data.
[0090] Specifically, the historical data obtained is the original electricity consumption data. In practical applications, due to some external reasons such as personnel and equipment, sometimes there will be a lack of data items generated by one or more fields, and sometimes there will be a large amount of information. An error in the field. Therefore, the process of preprocessing the data is required, and the preprocessing includes steps such as data cleaning.
[0091] In one implementation, see image 3 , in step S1, the preprocessing of the obtained historical data specifically includes:
[0092] Step S1.1: Data sampl...
Embodiment 2
[0145] This embodiment provides a device for predicting the risk of stealing electricity based on deep learning, please refer to Figure 8 , the device consists of:
[0146] A preprocessing module 301, configured to acquire historical data from actual application scenarios of on-site metering equipment, and preprocess the acquired historical data;
[0147] A feature extraction module 302, configured to extract climate features and electricity consumption features from the preprocessed data;
[0148] The daily electricity consumption pattern prediction module 303 is used to use the extracted climate characteristics and electricity consumption characteristics as training samples, and predict the user's daily electricity consumption pattern based on the training samples and the deep belief network DBN;
[0149] The abnormal electricity consumption rate detection module 304 is used to calculate the abnormal electricity consumption rate by using the preset analytic hierarchy proce...
Embodiment 3
[0184] Based on the same inventive concept, the present application also provides a computer-readable storage medium 400, please refer to Figure 9 A computer program 411 is stored thereon, and the method in Embodiment 1 is implemented when the program is executed.
PUM
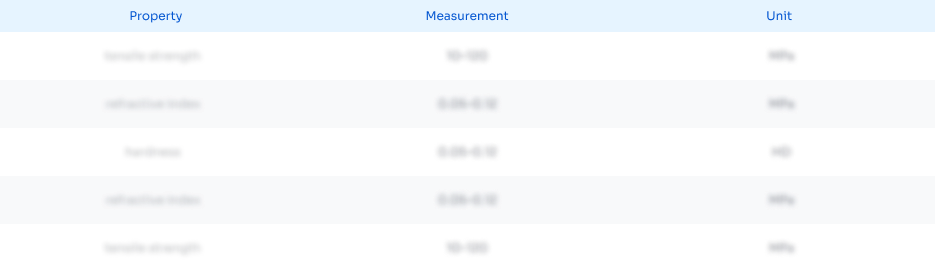
Abstract
Description
Claims
Application Information
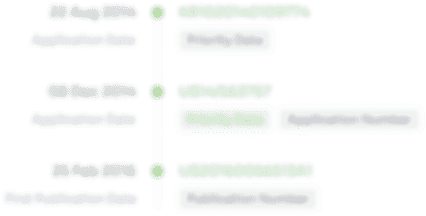
- R&D Engineer
- R&D Manager
- IP Professional
- Industry Leading Data Capabilities
- Powerful AI technology
- Patent DNA Extraction
Browse by: Latest US Patents, China's latest patents, Technical Efficacy Thesaurus, Application Domain, Technology Topic, Popular Technical Reports.
© 2024 PatSnap. All rights reserved.Legal|Privacy policy|Modern Slavery Act Transparency Statement|Sitemap|About US| Contact US: help@patsnap.com