Residual service life prediction method of complex equipment based on combined depth neural network
A deep neural network and life prediction technology, applied in neural learning methods, biological neural network models, neural architectures, etc., to achieve the effects of improving effective life, suppressing noise, and simple structure
- Summary
- Abstract
- Description
- Claims
- Application Information
AI Technical Summary
Problems solved by technology
Method used
Image
Examples
Embodiment Construction
[0054] The present invention will be further described below in conjunction with accompanying drawing and turbine engine data set as specific example:
[0055] like figure 1 As shown, the embodiment of the present invention uses a turbine engine as an example for illustration, specifically including the following steps:
[0056] This example uses the C-MAPSS dataset of the National Aeronautics and Space Administration (NASA) Forecast Data Warehouse to verify the effectiveness of the proposed method. This data set is simulated data obtained through simulation using the Commercial Modular Aerospace Propulsion System (C-MAPSS) developed by NASA. According to different operating states and failure modes, it can be further divided into 4 independent subsets, each subset contains a training set and a test set, and each subset contains engine operating data obtained through 21 sensors.
[0057] In the simulation program, the training set consists of sensor data records collected fr...
PUM
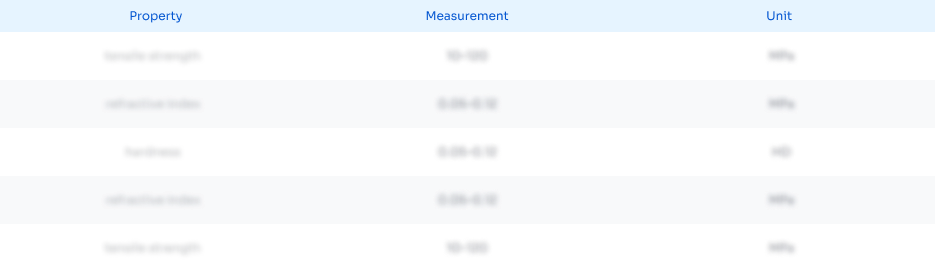
Abstract
Description
Claims
Application Information
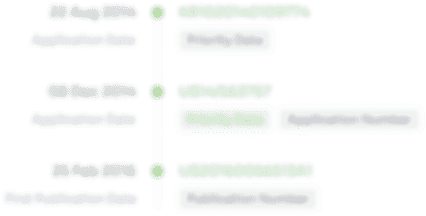
- R&D Engineer
- R&D Manager
- IP Professional
- Industry Leading Data Capabilities
- Powerful AI technology
- Patent DNA Extraction
Browse by: Latest US Patents, China's latest patents, Technical Efficacy Thesaurus, Application Domain, Technology Topic, Popular Technical Reports.
© 2024 PatSnap. All rights reserved.Legal|Privacy policy|Modern Slavery Act Transparency Statement|Sitemap|About US| Contact US: help@patsnap.com