Block-based robust tensor principal component analysis method
A principle component analysis and robust technology, applied in the field of image processing, can solve problems such as large noise points or abnormal points, rough processing of sparse components, etc., and achieve the effect of easy parameter setting, accurate and clear details
- Summary
- Abstract
- Description
- Claims
- Application Information
AI Technical Summary
Problems solved by technology
Method used
Image
Examples
Embodiment
[0104] In order to verify the effectiveness of the present invention, a color image denoising experiment was carried out in the present invention. Its running platform is MatLab R2016a, the processor is Intel 2.60GB i5-3230M, RMB is 8GB, and a notebook with Windows 10 system.
[0105] For space size N 1 ×N 2 RGB color image, the RGB color image is essentially a 3-dimensional tensor Each channel of a color image can be seen as Of a frontal slice. The image can be approximately reconstructed by a low-order matrix. Considering that t-SVD is a multi-linear extension of SVD, in this embodiment, a low tube rank tensor is used to approximate a color image.
[0106] Apply the RBTPCA method of the present invention to the color image denoising processing, and compare the performance with the existing IBTSVT method and RTPCA method:
[0107] Randomly select 50 color images for testing. For each color image, 10% of the pixels are randomly selected, which is randomly selected in the interva...
PUM
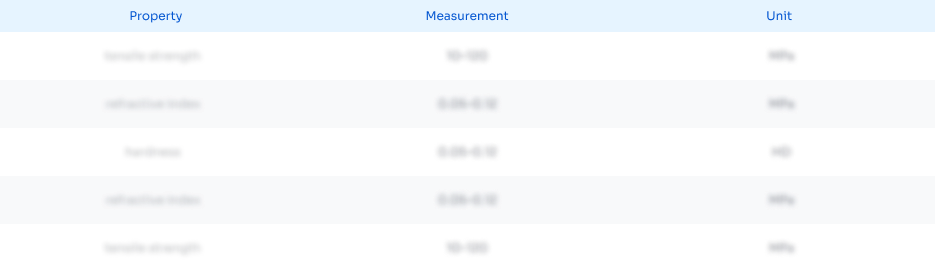
Abstract
Description
Claims
Application Information
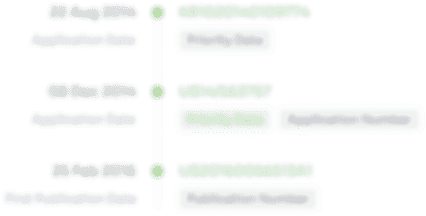
- R&D Engineer
- R&D Manager
- IP Professional
- Industry Leading Data Capabilities
- Powerful AI technology
- Patent DNA Extraction
Browse by: Latest US Patents, China's latest patents, Technical Efficacy Thesaurus, Application Domain, Technology Topic, Popular Technical Reports.
© 2024 PatSnap. All rights reserved.Legal|Privacy policy|Modern Slavery Act Transparency Statement|Sitemap|About US| Contact US: help@patsnap.com