A machine learning hyperparametric optimization system and method based on asynchronous Bayesian optimization
A machine learning and optimization method technology, applied in the field of machine learning hyperparameter optimization system, can solve the problems of reduced search efficiency, low efficiency, and inability to effectively deal with the big data environment, and achieve the effect of efficient hyperparameter optimization efficiency
- Summary
- Abstract
- Description
- Claims
- Application Information
AI Technical Summary
Problems solved by technology
Method used
Image
Examples
Embodiment Construction
[0036] The technical scheme of the present invention can be expressed as Figure 4 , mainly including: Bayesian optimization module, model parameter pool model, Kmeans clustering module, task scheduling module, and adaptive determination model parallelism module. Through the collaborative work of the above modules, the machine learning hyperparameter optimization method based on Bayesian optimization proposed by the present invention can be realized.
[0037] Among the above modules, the Bayesian optimization module:
[0038] The Bayesian optimization module is the basic technology of the present invention. This module mainly implements the Bayesian optimal method. The Bayesian optimization models the relationship between model evaluation indicators and parameter points, and can generate more meaningful parameter points. In the present invention, Bayesian optimization is responsible for generating candidate parameter points and receiving feedback information (parameter points...
PUM
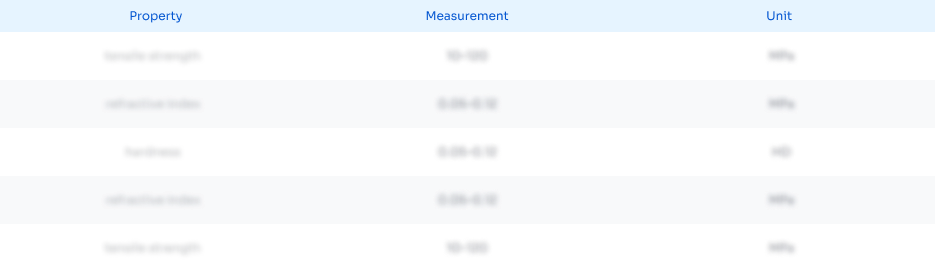
Abstract
Description
Claims
Application Information
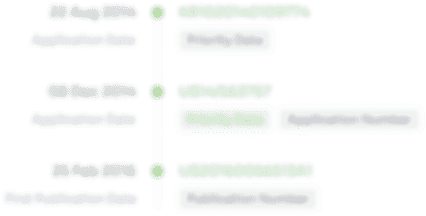
- R&D Engineer
- R&D Manager
- IP Professional
- Industry Leading Data Capabilities
- Powerful AI technology
- Patent DNA Extraction
Browse by: Latest US Patents, China's latest patents, Technical Efficacy Thesaurus, Application Domain, Technology Topic, Popular Technical Reports.
© 2024 PatSnap. All rights reserved.Legal|Privacy policy|Modern Slavery Act Transparency Statement|Sitemap|About US| Contact US: help@patsnap.com