Finger vein recognition method, apparatus and device
A recognition method, finger vein technology, applied in biometric recognition, character and pattern recognition, instruments, etc., can solve the problems of reduced recognition accuracy, reduced recognition speed, and large time consumption, so as to reduce the number of comparisons and ensure accuracy rate and speed, which is conducive to the effect of accurate comparison
- Summary
- Abstract
- Description
- Claims
- Application Information
AI Technical Summary
Problems solved by technology
Method used
Image
Examples
Embodiment 1
[0056] This embodiment proposes a finger vein identification method, please refer tofigure 1 , figure 1 This is a flowchart of the finger vein identification method provided in this embodiment; the method may include:
[0057] Step s110: Divide the received image to be matched into blocks to obtain several image blocks.
[0058] The received image to be matched may be divided into uniform blocks to facilitate subsequent feature extraction and splicing. This embodiment takes uniform division as an example.
[0059] It should be noted that the image to be matched before the block is a preprocessed image, and the process of preprocessing is not limited here. You can refer to the existing preprocessing process. For example, you can extract the ROI area of the collected biometric image, Angle correction, grayscale adjustment and size normalization, etc.
[0060] Step s120, perform SIFT feature extraction on several image blocks to obtain SIFT feature values of each image bloc...
Embodiment 2
[0085] The above embodiment does not limit the selection rules of candidate classes. In this embodiment, the overall identification process is introduced by taking the distance between the image to be matched and each cluster center as the candidate class selection condition as an example, which mainly includes the following steps:
[0086] The image to be matched is divided into 4 blocks on average, and SIFT features are extracted from each image block to obtain the SIFT feature values of the 4 image blocks.
[0087] Calculate the distance of 4 SIFT eigenvalue features to 10 class centers in the image library.
[0088] The class with the smallest distance between each image block and the 10 class centers is: the class with the smallest distance of image block 1 is the first class, the class with the smallest distance of image block 2 is the second class, and the class with the smallest distance of image block 3 is the first class The class with the smallest distance between i...
Embodiment 3
[0091] Based on the above embodiment, since the similarity between each image block and the cluster center may be calculated, there may be a large gap between the possible matching classes of each image block, and there may be more candidate classes obtained by simply analyzing and screening the distance of each image block. , the error is relatively large, in order to improve the accuracy, preferably, the screening of candidate classes may specifically include the following steps:
[0092] Step 1: Calculate the distance between each image block and each cluster center to determine the category of each image block;
[0093] Step 2: Encode each image block according to the category label of the category to which it belongs;
[0094] Step 3: Counting the coding of each image block in the image to be matched according to the image block splitting rule, and using the statistical result as the feature code of the image to be matched;
[0095] Step 4: Perform feature code matching ...
PUM
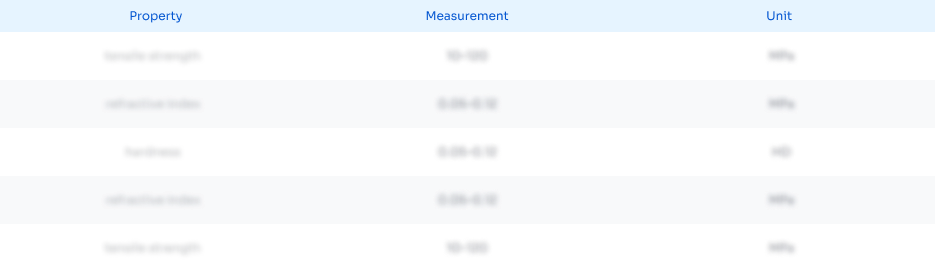
Abstract
Description
Claims
Application Information
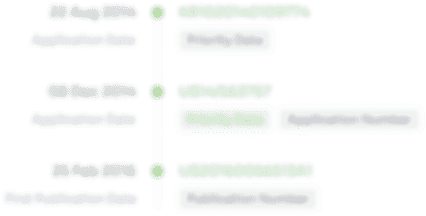
- Generate Ideas
- Intellectual Property
- Life Sciences
- Materials
- Tech Scout
- Unparalleled Data Quality
- Higher Quality Content
- 60% Fewer Hallucinations
Browse by: Latest US Patents, China's latest patents, Technical Efficacy Thesaurus, Application Domain, Technology Topic, Popular Technical Reports.
© 2025 PatSnap. All rights reserved.Legal|Privacy policy|Modern Slavery Act Transparency Statement|Sitemap|About US| Contact US: help@patsnap.com