A small sample target recognition method based on hybrid attribute learning
A technology of target recognition and attribute learning, which is applied in the field of small-sample target recognition based on mixed attribute learning, can solve problems such as poor discrimination ability, and achieve the effect of improving discrimination ability
- Summary
- Abstract
- Description
- Claims
- Application Information
AI Technical Summary
Problems solved by technology
Method used
Image
Examples
Embodiment Construction
[0034] The present invention is further analyzed below in conjunction with specific examples.
[0035] In this experiment, cats are used as the sample data set for training. Include steps such as figure 1 Shown:
[0036] Step (1), define semantic attributes and obtain auxiliary samples
[0037] Define semantic attributes according to the target to be learned, and at the same time obtain samples that contain the defined semantic attributes but are different from the target object as auxiliary samples. For example: using dogs as an auxiliary sample. The semantic attributes include, but are not limited to: four legs, two eyes, and whiskers, which are common to both dogs and cats.
[0038] Step (2), building a feature subnetwork
[0039] The feature sub-network is divided into two, one is used to extract the hidden attributes of the target training samples, and the other is used to extract the semantic attributes of the auxiliary samples.
[0040] The features of the first f...
PUM
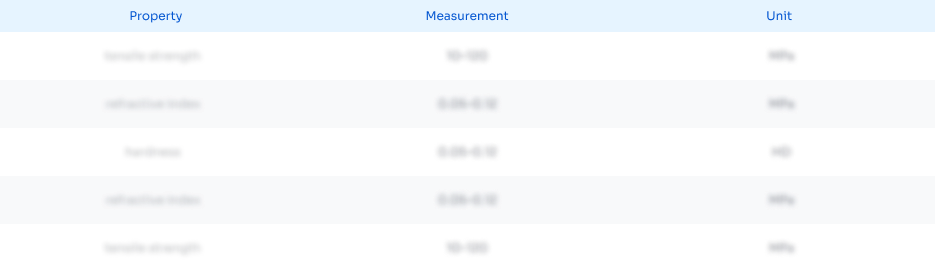
Abstract
Description
Claims
Application Information
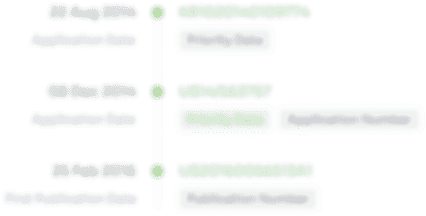
- R&D Engineer
- R&D Manager
- IP Professional
- Industry Leading Data Capabilities
- Powerful AI technology
- Patent DNA Extraction
Browse by: Latest US Patents, China's latest patents, Technical Efficacy Thesaurus, Application Domain, Technology Topic.
© 2024 PatSnap. All rights reserved.Legal|Privacy policy|Modern Slavery Act Transparency Statement|Sitemap