A wind speed prediction method and system based on a neighborhood gate long-short-term memory network
A long-term and short-term memory, wind speed prediction technology, applied in forecasting, weather condition prediction, biological neural network model, etc., can solve the problems such as the inability to accurately consider the wind speed factor structure, the inability to accurately consider the causal relationship, and the limited wind speed accuracy.
- Summary
- Abstract
- Description
- Claims
- Application Information
AI Technical Summary
Problems solved by technology
Method used
Image
Examples
Embodiment Construction
[0094] In order to make the objectives, technical solutions and advantages of the present invention clearer, the present invention will be further described in detail below in conjunction with embodiments. It should be understood that the specific embodiments described herein are only used to explain the present invention, but not to limit the present invention.
[0095] The present invention first adopts the method of "decomposition-dummy variable-pruning" to convert all types of causal relationship structures into a unified equivalent tree structure, and then establishes corresponding long and short-term memory based on neighborhood gates for the equivalent tree structure Network (NLSTM) to predict wind speed. The structure of NLSTM is the same as the equivalent tree structure, so it can accurately consider the causal relationship structure between factors.
[0096] figure 1 Shown is the overall flow chart of the wind speed prediction method of the neighborhood gate long short-...
PUM
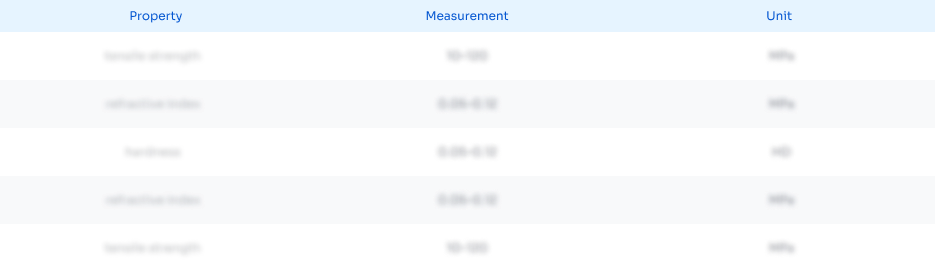
Abstract
Description
Claims
Application Information
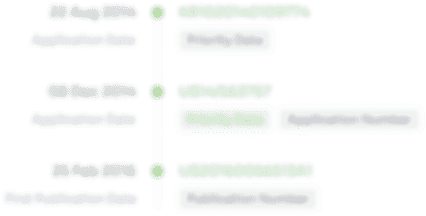
- R&D Engineer
- R&D Manager
- IP Professional
- Industry Leading Data Capabilities
- Powerful AI technology
- Patent DNA Extraction
Browse by: Latest US Patents, China's latest patents, Technical Efficacy Thesaurus, Application Domain, Technology Topic, Popular Technical Reports.
© 2024 PatSnap. All rights reserved.Legal|Privacy policy|Modern Slavery Act Transparency Statement|Sitemap|About US| Contact US: help@patsnap.com