Object-level classification sample automatic selection method for collaborative change detection
A technology of automatic selection and change detection, applied in the field of remote sensing, can solve the problems of high labor and material cost, and achieve the effect of improving efficiency and speed
- Summary
- Abstract
- Description
- Claims
- Application Information
AI Technical Summary
Problems solved by technology
Method used
Image
Examples
Embodiment 1
[0030] The main idea of the present invention is to find the "unchanged" ground objects on the two-phase remote sensing images, and based on this, establish the mapping relationship between the "unchanged" regions between the two phases of images, and use the mapping relationship to convert the previous image The sample information is migrated to the new image to realize the automatic migration and reuse of samples at different times.
[0031] The premise of the present invention is to obtain two phases of remote sensing images of the same research area, and the two phases of images are required to have similar spectral and spatial resolutions, that is, the optimal solution is that the two phases of images come from the same sensor, and the previous auxiliary remote sensing images have corresponding The sample or classification result information of . Specific steps are as follows:
[0032] 1) Multi-scale segmentation is performed on the image of the new time phase by means...
Embodiment 2
[0046] figure 1The main realization idea of the present invention is illustrated. The key steps of the present invention include mutual matching between remote sensing images, including spatial geometry matching and spectral radiation matching; multi-scale segmentation of new time-phase remote sensing images using the mean value shift method to extract ground objects; image matching between different time-phase images Meta-level change detection, using the spatial position relationship between "unchanged" pixels to establish the mapping of sample information between images; using the established mapping relationship to migrate sample class labels; The sample information migrated on the pixel constructs the "invariant" object and its sample label; the object sample of the new image is purified by setting a threshold, and the wrong sample is eliminated, and finally more reliable new sample data is obtained for subsequent classification. training process.
[0047] figure 2 ...
Embodiment 3
[0062] Taking the two phases of SPOT5 in Dongguan City as the test images, the automatic selection of samples was carried out through the method of the present invention, and the effect is as follows Figure 4 shown. It can be seen that new object-level samples have been established for garden land, grassland, construction land, water area, cultivated land, and wasteland in the image. Model training and automatic classification of ground objects can be carried out on the basis of sample establishment. The results are as follows: Figure 5 shown. After verification, its user accuracy is as follows: 89.6% for cultivated land, 92.6% for garden land, 68.91% for grassland, 59.1% for construction land, 69.2% for water area, 53.3% for wasteland, the overall accuracy is 80.6%, and the kappa coefficient is 0.7379. In this automatic sample selection process, manual visual collection is not relied on, and the accuracy of subsequent classification results based on automatically collecte...
PUM
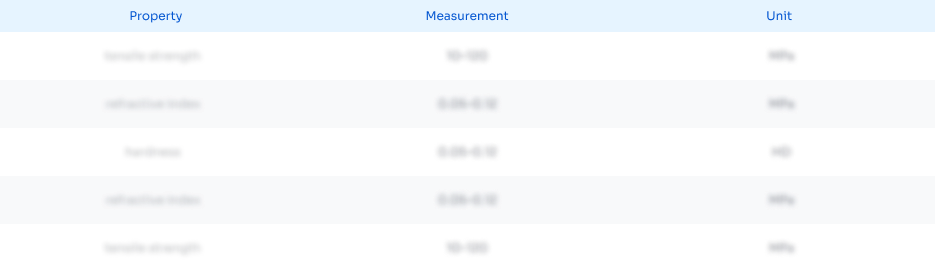
Abstract
Description
Claims
Application Information
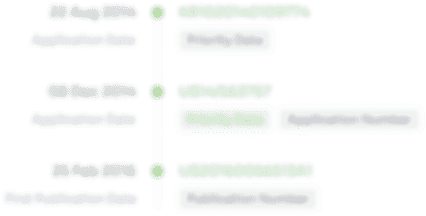
- R&D Engineer
- R&D Manager
- IP Professional
- Industry Leading Data Capabilities
- Powerful AI technology
- Patent DNA Extraction
Browse by: Latest US Patents, China's latest patents, Technical Efficacy Thesaurus, Application Domain, Technology Topic, Popular Technical Reports.
© 2024 PatSnap. All rights reserved.Legal|Privacy policy|Modern Slavery Act Transparency Statement|Sitemap|About US| Contact US: help@patsnap.com