L0-hinge loss function-based robust classification method
A technology of loss function and classification method, applied in the computer field, can solve the problem that the discreteness of training samples cannot be preserved
- Summary
- Abstract
- Description
- Claims
- Application Information
AI Technical Summary
Problems solved by technology
Method used
Image
Examples
Embodiment 1
[0058] The invention discloses a 0 - Robust classification methods for hinge loss functions such as figure 1 As shown, the method steps are as follows:
[0059] S1 obtains training set data
[0060] S2 calculates the kernel matrix K about the training sample, and makes the main diagonal element of the n-dimensional diagonal square matrix Y the training label;
[0061] S3 initializes parameter vectors u and v, penalty function penalty factor β;
[0062] S4 in the robust classifier l 0 - In the SVM model, u and v are solved alternately and iteratively through the block coordinate descent method to minimize the error of the objective function;
[0063] S5 as a robust classifier l 0 -The relative error of the objective function of the SVM model is less than the set threshold, so that the combination coefficient of the hyperplane parameter w Bias b=u n+1 , skip to S6; otherwise, increase penalty factor β, and skip to S4;
[0064] S6 outputs α, b;
[0065] S7 predicts t...
Embodiment 2
[0081] Experiment with real-life binary classification datasets.
[0082] The datasets used in the experiment are provided by the National Taiwan University LIBSVM website, and the datasets used are
[0083] Australian: Australian credit approval data set, a total of 690 samples, each sample has 14 attributes as features;
[0084] breast-cancer: A breast cancer database provided by William H. Wolberg of the University of Wisconsin-Madison Hospital in the United States, with a sample size of 683 and a feature dimension of 10.
[0085] Fourclass: The number of samples is 862, and the feature dimension is 2
[0086] German: German credit data set, which divides the credit risk of people through a set of characteristics, the number of samples is 1000, and the feature dimension is 24.
[0087] Sonar: The number of samples is 208, and the feature dimension of each sample is 60. Of these, 111 samples described sonar signals about metallic objects and 97 samples described sonar sig...
PUM
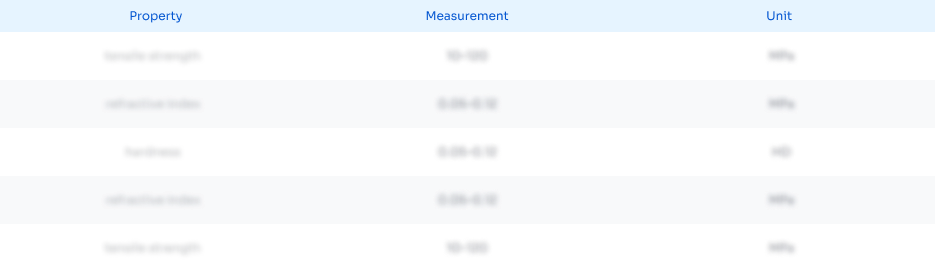
Abstract
Description
Claims
Application Information
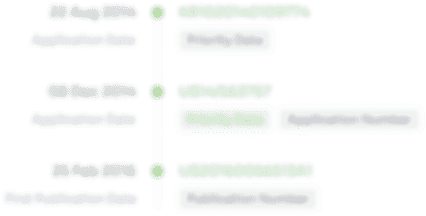
- R&D Engineer
- R&D Manager
- IP Professional
- Industry Leading Data Capabilities
- Powerful AI technology
- Patent DNA Extraction
Browse by: Latest US Patents, China's latest patents, Technical Efficacy Thesaurus, Application Domain, Technology Topic, Popular Technical Reports.
© 2024 PatSnap. All rights reserved.Legal|Privacy policy|Modern Slavery Act Transparency Statement|Sitemap|About US| Contact US: help@patsnap.com