A multi-instance multi-label learning method for safe city video surveillance applications
A technology of video monitoring and learning methods, which is applied in the field of multi-instance multi-label learning, and can solve the problems of internal connection of high-level features and large amount of calculation
- Summary
- Abstract
- Description
- Claims
- Application Information
AI Technical Summary
Problems solved by technology
Method used
Image
Examples
Embodiment Construction
[0031] In order to facilitate those of ordinary skill in the art to understand and implement the present invention, the present invention will be described in further detail below in conjunction with the accompanying drawings and embodiments. It should be understood that the implementation examples described here are only used to illustrate and explain the present invention, and are not intended to limit this invention.
[0032] Hundreds of millions of cameras in a city can collect a large amount of data every day. These data have no labels and no description information. The video surveillance at the place will be labeled as robbery, so city managers can use these labels to carry out centralized management, focus on rectification, and mark some potential high-risk areas for preventive treatment. Therefore, labeling urban traffic and security conditions is helpful. To promote urban management and the construction of a safe city. The present invention first extracts many high-...
PUM
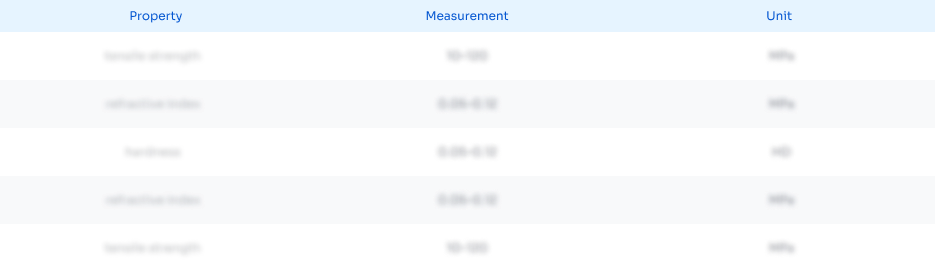
Abstract
Description
Claims
Application Information
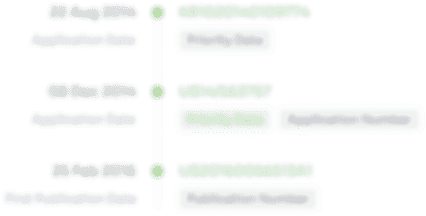
- R&D Engineer
- R&D Manager
- IP Professional
- Industry Leading Data Capabilities
- Powerful AI technology
- Patent DNA Extraction
Browse by: Latest US Patents, China's latest patents, Technical Efficacy Thesaurus, Application Domain, Technology Topic, Popular Technical Reports.
© 2024 PatSnap. All rights reserved.Legal|Privacy policy|Modern Slavery Act Transparency Statement|Sitemap|About US| Contact US: help@patsnap.com