Data distribution characteristic-based order-preserving learning machine
A technology of data distribution and learning machine, applied in the field of order-preserving learning machine, can solve the problems that the classification performance cannot be further improved, the relative relationship of various samples is ignored, and the characteristics of data distribution are not considered.
- Summary
- Abstract
- Description
- Claims
- Application Information
AI Technical Summary
Problems solved by technology
Method used
Image
Examples
Embodiment 1
[0088] Artificially generate five types of Gaussian distribution data sets, 40 samples of each type, and the center points of each type are (0,0), (6,6), (12,12), (18,18), (24,24 ), and the standard deviation is set to 2. Generate datasets such as figure 2 As shown, the direction vector obtained by RPLM-DDF is W, and the generated data is projected to W to obtain image 3 From the experimental results shown, it can be seen that the RPLM-DDF of the example has good separability and keeps the relative order of the samples unchanged.
Embodiment 2
[0090] The experimental data set uses the Iris standard data set. The dataset consists of 3 different types of irises, with a total of 150 samples. Each sample consists of five attributes: sepal length, sepal width, petal length, petal width, and type. Randomly select 60% of the Iris dataset as the training set, and the remaining 40% as the test set.
[0091] The effectiveness of RPLM-DDF is verified by comparative experiments with SVC (Support Vector Classification) and Naive Bayesian. The kernel function of RPLM-DDF algorithm adopts Gaussian kernel function. Use the grid search method to get the experimental parameters, ν is selected in {0.1,0.5,1,3,5,10}, σ is in The optimal experimental parameter is ν=0.5, SVC uses a Gaussian kernel function with a penalty factor C of 1. The Naive Bayesian algorithm does not set the prior probability, the experimental results are as follows Figure 4 shown. Depend on Figure 4 It can be seen that compared with SVC and Naive Bayes...
PUM
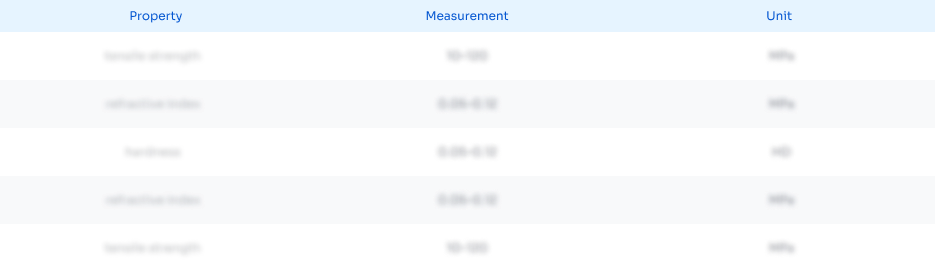
Abstract
Description
Claims
Application Information
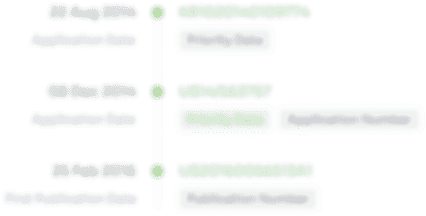
- R&D Engineer
- R&D Manager
- IP Professional
- Industry Leading Data Capabilities
- Powerful AI technology
- Patent DNA Extraction
Browse by: Latest US Patents, China's latest patents, Technical Efficacy Thesaurus, Application Domain, Technology Topic, Popular Technical Reports.
© 2024 PatSnap. All rights reserved.Legal|Privacy policy|Modern Slavery Act Transparency Statement|Sitemap|About US| Contact US: help@patsnap.com