24-hour electric power load prediction method
A power load, 24-hour technology, applied in the direction of forecasting, neural learning methods, data processing applications, etc., can solve the problem that there is no comprehensive analysis considering temperature or weather, inaccurate load forecasting, forecasting accuracy and practicality cannot be satisfied at the same time Requirements and other issues to achieve the effect of improving prediction accuracy and good prediction effect
- Summary
- Abstract
- Description
- Claims
- Application Information
AI Technical Summary
Problems solved by technology
Method used
Image
Examples
Embodiment Construction
[0018] The technical solutions in the present invention will be further described below in conjunction with the accompanying drawings and embodiments.
[0019] As shown in the figure, the present invention proposes a 24-hour power load forecasting method, comprising the following steps;
[0020] a. Data collection and preprocessing: Collect power grid data and weather data to form the original evaluation matrix Mnm, and perform linear transformation and normalization processing on the original evaluation matrix Mnm to obtain the matrix Snm, and carry out the data of each index according to the characteristic entropy weight Standardize the processing to get the corresponding weight value;
[0021] b. The effective features calculated by the feature entropy weight, combined with the window selection method, select the 24-hour electricity load within a fixed period of time as the input data for DBN network training;
[0022] c. According to the characteristics and distribution i...
PUM
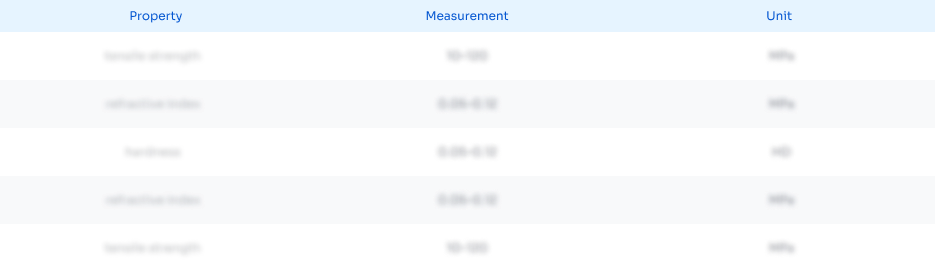
Abstract
Description
Claims
Application Information
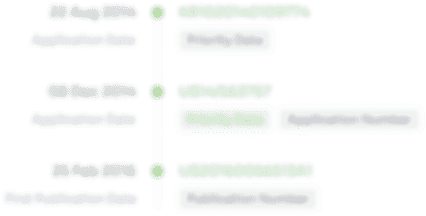
- R&D Engineer
- R&D Manager
- IP Professional
- Industry Leading Data Capabilities
- Powerful AI technology
- Patent DNA Extraction
Browse by: Latest US Patents, China's latest patents, Technical Efficacy Thesaurus, Application Domain, Technology Topic, Popular Technical Reports.
© 2024 PatSnap. All rights reserved.Legal|Privacy policy|Modern Slavery Act Transparency Statement|Sitemap|About US| Contact US: help@patsnap.com