Image restoration method based on convolutional neural network with symmetric cross layer connection
A technology of convolutional neural network and neural network, applied in the field of image restoration
- Summary
- Abstract
- Description
- Claims
- Application Information
AI Technical Summary
Problems solved by technology
Method used
Image
Examples
Embodiment 1
[0095] This embodiment describes image denoising, including the following parts:
[0096] 1. First, on the BSD natural image data set, a total of 500,000 image blocks of 50*50 are randomly intercepted, and Gaussian noise with a mean value of 0 and a standard deviation of σ is added to the image (σ takes 10, 30, 50, and 70 respectively Four experiments were performed). Normalize the image after adding noise and the image without adding noise. Thus, a data set with a size of 500,000 is obtained, in which 450,000 image blocks are used as the training set and 50,000 as the verification set.
[0097] 2. Construct a 30-layer convolutional neural network with cross-layer connections, use the ADAM algorithm to train on the constructed data set, and set the learning rate to 1e-4 uniformly, and use the verification set to verify the parameter pre-training effect in each training round. Finally, a converged network is obtained.
[0098] 3. Test on the original image of the BSD200 test...
Embodiment 2
[0103] This embodiment describes image super-resolution, including the following parts:
[0104] 1. First, on the BSD natural image data set, a total of 500,000 image blocks of 50*50 are randomly intercepted. For each image block, the linear interpolation algorithm is used to shrink by s times (s is 2, 3 and 4 for three experiments), On the basis of the reduced image, a low-resolution image with the same size as the original image but with low definition is obtained by s-fold enlargement. Combine the low-resolution image with the original image to obtain a data set with a size of 500,000, of which 450,000 image blocks are used as the training set and 50,000 as the verification set.
[0105] 2. Construct a 20-layer convolutional neural network with cross-layer connections, use the ADAM algorithm to train on the constructed data set, and set the learning rate to 1e-4 uniformly, and use the verification set to verify the parameter pre-training effect in each training round. Fina...
PUM
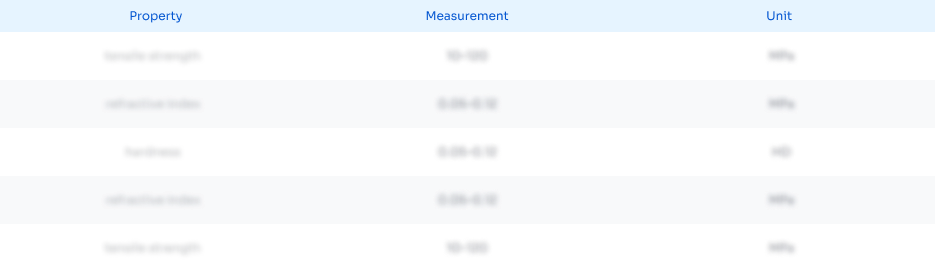
Abstract
Description
Claims
Application Information
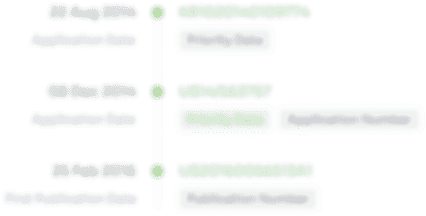
- R&D Engineer
- R&D Manager
- IP Professional
- Industry Leading Data Capabilities
- Powerful AI technology
- Patent DNA Extraction
Browse by: Latest US Patents, China's latest patents, Technical Efficacy Thesaurus, Application Domain, Technology Topic, Popular Technical Reports.
© 2024 PatSnap. All rights reserved.Legal|Privacy policy|Modern Slavery Act Transparency Statement|Sitemap|About US| Contact US: help@patsnap.com